
A Comparative Study of Predictive Coverage Based on Traditional Chinese Medicine Boinformatics Database and Analytics Tool Choices
Ⓒ The Society of Pathology in Korean Medicine, The Physiological Society of Korean Medicine
Abstract
As the demand for scientific validation of traditional medicine increases, network pharmacology research utilizing various bioinformatics databases has been actively conducted. However, clear guidelines on how differences in prediction outcomes may vary depending on the choice of database are lacking. This study aims to compare two major bioinformatics databases for herbal medicine (TCMSP, BATMAN-TCM) to analyze how the choice of database influences prediction results and to propose a more effective analytical approach. We compared the prediction results among three scenarios composed of different combinations from two TCM bioinformatics databases (TCMSP, BATMAN-TCM) and one analytics tool (DAVID) with an example herb, licorice. The gene ontology terms (GO-term) and Kyoto encyclopedia of genes and genomes (KEGG) pathway terms were compared and prediction ranges of two TCM bioinformatics databases for disease were compared against to disease list derived from in vivo study literatures of licorice written in the last 10 years. The three scenarios showed different trends in enrichment analysis with GO-term and KEGG pathway term. Scenario A showed a trend of cancer (apoptotic process, p=1.80E-32; response to hypoxia, p=8.90E-31, regulation of apoptotic process, p=2.30E-30, regulation of programmed cell death, p=6.40E-30, pathways in cancer, p=6.10E-36) whereas other two scenarios showed similar trend of neurotransmission (regulation of ion transport, p=1.00E-82, cell-cell signaling, p=9.16E-85, neuroactive ligand-receptor interaction, p=1.66E-37). The 58% and 52% of diseases derived from in vivo experiment study literatures were predicted by each TCM bioinformatics database. These results indicate that differences in target lists predicted from different databases lead to differences in enrichment analysis, which in turn leads to differences in disease prediction coverage. Thus, using a merged target list predicted by at least two TCM bioinformatics databases may provide more unbiased, complete, and wider range of results.
Keywords:
Bioinformatics, Network pharmacology, TCMSP, BATMAN-TCM, Traditional Korean medicine (TKM), LicoriceIntroduction
Unlike Western medicine, the practitioners in traditional Korean medicine (TKM) prescribe herbal medicines with more than one herb that contains numerous ingredients1). In addition, an efficacy of an herbal formula has been explained by the inherent, combined efficacies of each herb—not by ingredient—and by the interactions between herbs from the perspective of the TKM theory2). However, due to the changing trends in traditional medicines in South Korea, this traditional approach has lost its credibility from both groups of patient and practitioner3,4). This trend is considered to be a public request for scientific validation of the possibility of ineffectiveness, low efficacy, or potential risk, even thought traditional prescription formulation principles of herbal medicine are well established5).
Verifying efficacy of herbal medicine via clinical trials needs relatively longer period of time and has low applicability to the vast number of herbal formulas described in old literatures of TKM6). In addition, many clinicians change composition of an herbal formula by adding or removing one or more herbs from an original composition, because each herb has own link to specific symptom or syndrome7). Most TKM practitioners change herbs in an herbal formula according to changes of patient’s symptoms, however this aspect could not have been considered in the clinical trial setting.
Network pharmacology is developed based on systems biology to design polypharmacy study and to discover candidate ingredients or targets by predicting the association between ingredients, target proteins, and disease data8). The “multi-ingredient to multi-target” prediction—main concept of network pharmacology—has been considered a new method that can help validate herbal medicine or TKM theories because of similarity to the efficacy theory of herbal formulas9). Many researchers have been actively using the network pharmacology in recent herbal medicine studies. Analysis of TKM using network pharmacology has the advantage of increasing the success rate of research through prediction before conducting new research with an herb or an herbal formula10,11).
A number of network pharmacology databases based on herbal medicine data have been developed; i.e., Traditional Chinese Medicine Systems Pharmacology database and analysis platform (TCMSP, https://old.tcmsp-e.com/tcmsp.php)12); Bioinformatics Analysis Tool for the Molecular mechanism of Traditional Chinese Medicine 1.0 (BATMAN-TCM 1.0, http://bionet.ncpsb.org.cn/batman-tcm/index.php/Home/Index/index)13); and Traditional Chinese Medicine Integrative Database (TCMID, http://47.100.169.139/tcmid/)14). The analysis process of herbal medicine-based network pharmacology is as follows. First, deriving ingredients consisting of an herb from TCM bioinformatics database. Second, deriving targets predicted from the ingredient list by TCM bioinformatics database. Thrid, predicting a mechanism of efficacies or related diseases from the target list by interpreting prediction results15-17). TCMSP and BATMAN-TCM databases are widely used to conduct a network analysis of each herb and herbal formula18-20). However, many comparative studies seemed to be limited to the comparison of the number of herbs, ingredients, targets, and diseases, or the main goal of the study was not the comparison of prediction difference related to the characteristics of databases with an example herb.
Licorice root is the most frequently used herb (27%) out of approximately 100,000 herbal formulas in traditional Chinese medicine21), which came from a variety of Glycyrrhiza species; i.e., Glycyrrhiza uralensis Fisch.; Glycyrrhiza glabra L.; Glycyrrhiza inflata Batalin; Glycyrrhiza aspera Pall.; Glycyrrhiza yunnanensis P.C.Li; Glycyrrhiza squamulose Franch., etc.. Licorice root, a qi-tonifying herb, is known to tonifies spleen, moistens dryness of lung, removes toxins, and coordinates with many other herbs in an herbal formula according to TKM theory22). Traditional indications for licorice root are varied; i.e., cough; sore throat; thirst; fatigue; erectile dysfunction; shortness of breath; pyogenic infections and resulting ulcers and abscesses; fever induced by deficient state; fright palpitation; irritancy; epilepsy; abdominal distension; forgetfulness; painful urination with blood of women; and lower back pain of women23). Among twenty-five representative candidate herbs, licorice was selected because of the highest numbers of ingredient derived—92 and 75—both from TCMSP and BATMAN-TCM. Another representative herb, ginseng was excluded owing to a big gap of the number of ingredients between databases with 22 and 112.
Many network pharmacology research papers do not seem to clearly present the protocols they used for their analysis or the reasons for choosing the databases or tools they used. This aspect has the potential to bias the interpretation of prediction results according to the databases or tools chosen. Thus, we aimed to explore how different the prediction results are shown between two popular TCM bioinformatics databases—TCMSP and BATMAN-TCM 1.0—designed by different prediction algorithms12,13). At the same time, we aimed to propose a more effective analysis approach by showing the difference in the prediction results between the two TCM bioinformatics databases.
In this study, we conducted brief comparison of the algorithmic difference between the two TCM bioinformatics databases with published literatures, and compared the actual prediction results from one example herb, licorice. Fig. 1. shows the overall process of this study.
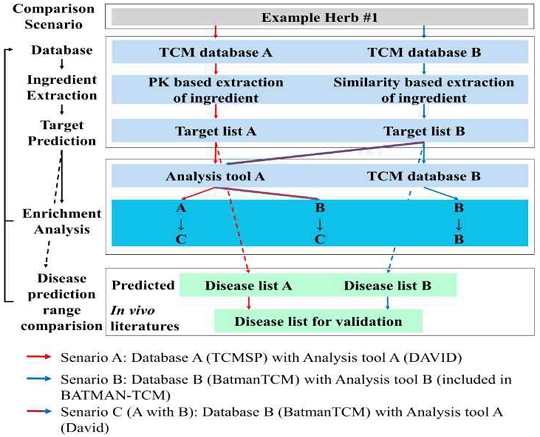
Overall process of study. The database for annotation, visualization, and integrated discovery (DAVID) was not used for disease prediction range comparison, and the disease lists collected from TCMSP and BATMAN-TCM are used for comparison against disease list collected from in vivo study literatures. Dotted arrows are for visibility purpose and have the same meaning as solid arrows; Abbreviations: PK, pharmacokinetics, TCM, traditional Chinese medicine
Materials and Methods
1. Comparison of prediction results using licorice
We derived a list of representative medicinal herbs from a textbook for medicinal herbs used in the current College of Korean Medicine curricula. We input ingredients on the list to TCMSP and BATMAN-TCM 1.0 database. BATMAN-TCM 2.0 (http://bionet.ncpsb.org.cn/batman-tcm/#/home) were not included in this study which was updated in October 2023. TCMID was excluded because of inaccessibility. Subsequently, we screened one herb with a sufficient number of ingredients from both databases to conduct a reliable analysis.
We compared prediction results from three different combinations of bioinformatics databases and analytics tools; enrichment analysis with DAVID using a target list predicted by TCMSP (DAV-SP: 1st scenario); enrichment analysis with DAVID using a target list predicted by BATMAN-TCM (DAV-BAT: 2nd scenario); and enrichment analysis with BATMAN-TCM using a target list predicted by BATMAN-TCM (BAT-BAT: 3rd scenario).
In TCMSP, an ingredient list was derived based on criteria of oral bioavailability (OB) ≥ 30% and drug-likeness (DL) ≥ 0.18, which are parameters reflecting the absorption, distribution, metabolism, and excretion (ADME) of an ingredient. In BATMAN-TCM, an ingredient list was derived based on a cut-off score of ≥ 20. We collected predicted target lists related to each ingredient by both TCM databases and converted full names of targets only from TCMSP to official gene symbols via UniProt database (https://www.uniprot.org/). BATMAN-TCM provides targets with official gene symbols. The ingredient-target networks were visualized and analyzed via Cytoscape software (ver. 3.9.1). The ingredients and targets were arranged by highest degree in the network.
2. Comparison of predictions from the different scenarios
The enrichment analysis of gene ontology term (GO-term) and Kyoto Encyclopedia of genes and genomes (KEGG) pathway term were compared to predict the biological action of an herb in the human body. Since BATMAN-TCM provides the enrichment analysis of GO-term, KEGG pathway and disease prediction results within the database (which TCMSP doesn’t provide), using BATMAN-TCM alone from predicting targets to analyzing results was also considered the third scenario.
We inputted the whole target lists from each database without top-10 priority adjustment into the DAVID, conducted enrichment analysis, and collected the top-10 GO-terms and KEGG pathway terms in a lowest order at the level of adjusted p-value less than 0.05. The top-10 enriched GO-terms and KEGG pathway terms related to biological process were compared between the three scenarios. The ‘GO-term_5’ result from DAVID was used for analysis because it had the highest specificity than other options. The top-10 enriched GO-terms and KEGG pathway terms were interpreted with reference to several databases, such as Gene Ontology Resource (https://geneontology.org/) and KEGG database (https://www.genome.jp/kegg/pathway.html).
3. Comparison of prediction range for diseases with in vivo study literatures
Prediction range for disease by the three scenarios were compared against disease list collected from in vivo study literatures. In PubMed (https://pubmed.ncbi.nlm.nih.gov), using the search term “licorice”, in vivo study literatures including clinical trial, case report, and animal study written in English over the past ten years were collected. Studies including herbs other than licorice were excluded. All diseases names were classified and unified by checking against international classification of diseases 11th (ICD-11) version code. The disease names not recognized as official disease names were excluded. Clinical trials including herbs other than licorice and animal studies without specific disease name were also excluded. We derived and listed diseases predicted from the whole target lists of TCMSP and BATMAN-TCM without Top-10 priority adjustment, respectively. Similar disease names were unified.
Results
1. Algorithmic differences between two databases
Although both TCM bioinformatics databases have common features in constructing datasets and utilizing existing Food and Drug Administration (FDA)-approved drug information, the main difference was present in the method of analysis. TCMSP predicts the ingredient-target interactions based on molecular and ligand structural pattern analysis, whereas BATMAN-TCM predicts the binding potential based on a similarity comparison with putative ingredients. In addition, TCMSP uses the self-developed artificial intelligence (AI) called SysDT, and BATMAN-TCM uses a similarity calculation formula12,13,24). SysDT predicts a target protein or ligand for a given ligand or target protein without initially setting a special similar dataset. The target proteins were selected based on their structural and physicochemical values, and predictions were made based on the extraction of conserved patterns from interaction vectors containing both the target protein and the encoding vector of the corresponding ligand24). Similarity scores between the candidate ingredient and the similar ingredient and between the candidate target protein and the similar protein selected from the standard dataset were calculated. The ingredient – ingredient similarity scores consisted of six different scores: two chemical structure similarity scores, a side-effect similarity score from the side effect resource database (SIDER), the Anatomic Therapeutic Chemical (ATC) classification system, drug-induced gene expression, and text mining scores. Each similarity score was correlated using the minimum redundancy maximum relevance method, and eight features with a high correlation were used in the predictive model. Finally, the prediction score is calculated using the maximum likelihood ratios for the eight features13). Therefore, it is speculated that these differences may affect the reliability and accuracy of the prediction results.
2. Comparison of ingredient and target lists of licorice
Fig. 2. shows the numbers and proportions of ingredients and targets derived from TCMSP and BATMAN-TCM. In TCMSP, from the total 281 ingredients, 92 ingredients were derived based on the OB and DL. Four ingredients without a predicted target were excluded; licorice glycoside E; glycyroside; glyuranolide; and 18α-hydroxyglycyrrhetic acid. Eighty eight ingredients were identified and 219 targets were identified from 1,657 ingredient-target pairs. In BATMAN-TCM, from the total 172 ingredients, 125 ingredients were derived after fifty ingredients without a predicted target were excluded. Seventy five ingredients were identified and 691 targets were identified from 1,593 ingredient-target pairs. Table 1 shows the ingredients and targets with the top-10 highest degree in ingredient-target network. The number of common ingredients between TCMSP and BATMAN-TCM changed from 49 (16%) to 7 (4.5%) after exclusion explained above. The seven common ingredients were glycyrol, glycyrin, isoglycyrol, licocoumarone, liquiritin, isotrifoliol, and 3’-methoxyglabridin. Among the top-10 ingredients, no common ingredients between TCMSP and BATMAN-TCM were found. The top-10 ingredients of TCMSP include quercetin and kaempferol whereas the top-10 ingredients of BATMAN-TCM include glycyrrhetic acid and glycyrrhetinic acid, which are a same ingredient. The number of common targets from the total target list between TCMSP and BATMAN-TCM was 79 (9.5%). Among the top-10 targets, common targets were AR (androgen receptor) and ESR1 (estrogen receptor 1).

The percentages of common ingredients or targets between TCMSP and BATMAN-TCM with different trends of ingredient-target networks. (A) Ingredients of the final list. (B) Targets from total lists. (C) Targets with top-10 highest degree in the ingredient-target network. (D) Difference of ingredient-target networks between TCMSP and BATMAN-TCM.
Fig. 2. shows the difference between the visualized networks of ingredient-target between TCMSP and BATMAN-TCM. The network of TCMSP tends to have a lot more ingredients connected to one target, whereas the network of BATMAN-TCM tends to have a lot more targets connected to one ingredient. (Fig. 2)
3. Comparison of predicted enrichment analysis of licorice
The top-10 enriched GO-terms showed different trends among three different scenarios of tools; DAV-SP scenario showed a trend of response and process of cell to stimulus including apoptosis (cellular response to organic cyclic compound; cellular response to lipid; cellular response to organonitrogen compound; response to steroid hormone; response to hypoxia; apoptotic process; regulation of apoptotic process; and regulation of programmed cell death); DAV-BAT scenario showed a trend of cell signaling and homeostasis (regulation of ion transport; cation transport; positive regulation of transport; ion transmembrane transport; regulation of transmembrane transport; regulation of ion transmembrane transport; ion homeostasis; cellular chemical homeostasis; and trans-synaptic signaling); and BAT-BAT scenario showed an ambiguous trend. No common GO-terms among the three combinations were shown from the top-10 results. Table 2 shows the top-10 enrichment analysis for biological process of GO-terms.
The top-10 enriched KEGG pathway terms showed different trends between three different scenarios; DAV-SP scenario showed a trend of cancer (pathways in cancer; prostate cancer; receptor activation-chemical carcinogenesis; pancreatic cancer; bladder cancer; and herpesvirus infection associated Kaposi sarcoma) and heart (Fluid shear stress and atherosclerosis; lipid and atherosclerosis; and AGE-RAGE signaling pathway in diabetic complications); DAV-BAT scenario showed a trend of neurotransmission and heart (neuroactive ligand-receptor interaction; calcium signaling pathway; cAMP signaling pathway; Cholinergic synapse; adrenergic signaling in cardiomyocytes; fluid shear stress and atherosclerosis; and oxytocin pathway). BAT-BAT scenario also showed a trend of neurotransmission and heart (neuroactive ligand-receptor interaction; calcium signaling pathway; cardiac muscle contraction; pathway of cyclic GMP to PKG signaling in cell; cholinergic synapse; and adrenergic signaling in cardiomyocytes). No common KEGG pathway terms between the three scenarios were shown from the top-10 results. The common KEGG pathway terms between DAV-SP and DAV-BAT scenarios were pathway in cancer, fluid sheer stress and atherosclerosis. The common KEGG pathway terms between DAV-BAT and BAT-BAT scenarios were neuroactive ligand-receptor interaction, calcium signaling pathway, glutathione metabolism, cholinergic synapse, and adrenergic signaling in cardiomyocytes. No common KEGG pathway terms were shown between DAV-SP and BAT-BAT scenarios. Table 3 shows the top-10 enriched KEGG pathway terms.
4. Comparison of recapitulation range of disease against to in vivo study literatures
In the process of categorizing and unifying disease names to ICD-11 codes, 25 (TCMSP) and 35 (BATMAN-TCM) diseases were excluded. Among 113 in vivo studies, 6 clinical trials including herbs other than licorice and 9 animal studies without specific disease name were excluded. Fifteen and 21 similar disease names in each list from TCMSP and BATMAN-TCM were unified.
TCMSP recapitulated 243 diseases whereas BATMAN-TCM recapitulated 391 diseases. Sixty two diseases were derived from the 98 in vivo study literatures consisted of 23 clinical trials, 11 case reports, and 64 animal studies. The number of common diseases between TCMSP and BATMAN-TCM was 184. The number of unique diseases was 59 for TCMSP (24%) and 207 for BATMAN-TCM (53%). Among total diseases recapitulated, the disease classifications with the highest frequency were as follows; Neoplasms; circulatory system; symptoms, signs or clinical findings, not elsewhere classified; nervous system; endocrine, nutritional or metabolic; and mental, behavioral or neurodevelopmental disorders. The diseases derived from literatures with highest frequency were as follows; colorectal cancer; hepatic cancer; caries; diabetes; liver injury; hypertension; severe hypokalemia; obesity, etc.
Among the 62 diseases derived from in vivo study literature, 26 diseases were recapitulated by TCMSP and 32 diseases were recapitulated by BATMAN-TCM. Among the recapitulated diseases, 2 diseases (endometrial carcinoma and lung cancer) were recapitulated by TCMSP alone, and 8 diseases (allergic rhinitis, unspecified; colitis; gastric cancer; hypotension; neutrophil-mediated liver injury; osteosarcoma; pulmonary hypertension; and type I hypersensitivity) were recapitulated by BATMAN-TCM alone. Twenty-eight diseases were not recapitulated by TCMSP and BATMAN-TCM. Fifty five percent of diseases were recapitulated by two databases among the diseases derived from in vivo study literatures.
Discussion
The popular bioinformatics databases for network analysis of herbal medicines, TCMSP and BATMAN-TCM, use different algorithms for target prediction. The main difference was whether they are focusing on molecular and ligand structural pattern analysis or on a comparison of the similarities12,13,24). Since the main algorithmic difference appears in ingredient-target prediction process, we expected that prediction result from same single herb, licorice, would be different between them. In the analysis using licorice, a representative herb in TCM, the first difference was found in the ingredient-target network. Only seven common ingredients and 79 targets were found between TCMSP and BATMAN-TCM. This heterogeneity of the predicted targets between two databases would inevitably lead to different prediction results. In addition, TCMSP revealed a phenomenon of over-concentrated prediction to quercetin and kaempferol, which has been pointed out as a limitation of TCM network analysis thus far25). Whereas, BATMAN-TCM seems to be less affected by this phenomenon. This study also found that quercetin and kaempferol are among the top-10 ingredients in TCMSP. The top-10 ingredients in BATMAN-TCM included glycyrrhetic acid and glycyrrhetinic acid, which are the main active ingredients in licorice for treatment of diseases and are also responsible for the side effect of pseudoaldosteronism26,27). Among the top-10 targets of TCMSP and BATMAN-TCM, the common targets were AR and ESR1, where AR is androgen receptor and ESR1 is estrogen receptor. This result is consistent with the fact that licorice has been studied for its effects on sex hormones28).
The three scenarios from different combinations of databases and a tool showed different trends in enrichment analyses of GO-terms and KEGG pathway terms. DAV-SP scenario showed a result related to cancer whereas DAV-BAT and BAT-BAT combinations both using the same target list predicted by BATMAN-TCM showed a similar trend related to neurotransmission. Of the top-10 ingredients in TCMSP, quercetin and kaempferol have antioxidant, anti-inflammatory, and anti-cancer properties, which are considered relevant to the cancer-related result29,30). All three scenarios showed common association to heart. Licorice’s effects on the heart have also been well studied including severe side effects31).
As we mentioned earlier, TCMSP seems to be biased toward cancer-related prediction. This study’s result from DAV-SP scenario also showed similar cancer-related prediction results. BAT-BAT scenario showed an ambiguous GO-term trend. Similar prediction results of enrichment analysis were shown between DAV-BAT and BAT-BAT. From this result, we may infer that the main factor contributing to the difference in prediction trends lies in the target list, other than the tool for analysis such as DAVID. Since the enrichment analysis process used only the two different target lists of licorice derived from each database, the differences in the target lists influenced the analysis process, resulting in the tendency toward different pathways. The TCMSP ingredient-target list dataset is constructed by mapping target proteins from DrugBank and ingredient datasets, and it is validated by the herbal ingredient target (HIT) database, which is bases on the latest abstracts from PubMed13). In contrast, BATMAN-TCM constructs its target list based on a ingredient dataset similar to FDA-approved ingredients. These differences in the foundational datasets and the algorithms used to construct the target lists are considered to have led to the tendency toward different pathways results. These differences in tendencies are encompassed within the already studied pharmacological actions of licorice, which has been shown to possess inhibitory effects on various cancer cells, including cervical, breast, liver, coon, pancreatic, and prostate cancers. In addition, licorice exibits anti-inflammatory and immunomodulatory effects which have close relationship with anti-tumor activity. Licorice acts similarly to adrenal cortex hormones, and has been researched for its memory-enhancing and neuroprotective properties32,33). Therefore, the differences between the databases described above appear to reflect tendencies in different directions within the scope of previously studied results. The most frequent diseases commonly predicted by two databases were neoplasm; followed by circulatory system; symptoms, signs or clinical findings; and nervous system. The diseases collected from the in vivo study literatures that were not predicted by the bioinformatics databases were as follows; 60% not predicted by TCMSP; 48% not predicted by BATMAN-TCM; and 45% not predicted by both databases. In addition, among the 24 diseases commonly predicted by TCMSP and BATMAN-TCM, three traditional indications of licorice similar to the modern disease names were found; epilepsy (epileptic seizures); forgetfulness (Alzheimer’s disease); and painful urination with blood and lower back pain of women (endometriosis).
TCMSP seems to have advantages of including different protein families within the prediction range and allowing researchers to adjust pharmacokinetic parameters such as OB and DL freely. BATMAN-TCM seems to have advantages of embedding real-world features such as anatomic therapeutic chemical (ATC) classification system and side effect data involved in the prediction algorithm. In addition, BATMAN-TCM provides prediction pages such as GO-term, KEGG pathway, and disease, all in one with P-value. Meanwhile, TCMSP does not provide a P-value in prediction results, which induces researchers to use other tools for analysis, such as DAVID. TCMSP showed an over-concentrated tendency toward specific ingredients and cancer-related disease prediction. BATMAN-TCM does not provide a specific explanation for various cut-off scores from 10 to 1,000.
Analyzing herb with different databases will result in different predicted targets, and therefore different predicted GO-terms, KEGG pathway terms and diseases. If the commonalities between the predicted ingredient or target lists from different databases are minimal, resulting in high heterogeneity, the expected effects of a single herb may appear fragmented and predicted separately. Therefore, when aiming to rigorously compare differences between databases, it is recommended to verify the extent to which the ingredient or target lists share commonalities above a certain threshold before proceeding with the study. Another option would be to merge predicted ingredient or target lists from at least two TCM bioinformatics databases, which could lead to unbiased, complete, and wider range of results.
This study has a few limitations. First, the differences between two TCM bioinformatics databases may not be explained enough because we only one herb is used for analysis. Second, we were unable directly verify the impact of differences of prediction algorithms on differences in actual prediction results. Thrid, we were unable to distinguish between licorice-treated and licorice-induced disease names from the predictions results. Fourth, the analysis to determine whether the observed differences in prediction results are statistically significant has not been performed. Fifth, the validation process of comparing the database predictions with actual biological experimental results has not been performed. Sixth, the list of diseases derived from a decade of in vivo studies was not enough to be considered as real-world data for verification of disease prediction results. Lastly, the high heterogeneity of target lists between databases made it inadequate to compare their functionality and predictive capabilities under the same conditions. Therefore, further study needs to be conducted using various herbs or herbal formulas with larger-scale real world dataset with the statistical significance and the experimental validation process.
The significance of this study is that we explored the process and reasons for the difference in prediction results compared to previous studies that simply analyzed the difference between prediction results. This study may be helpful to researchers who try to discover candidate ingredients from herbal medicine, to understand the mechanisms of action of herbal medicine, or to validate TKM theories.
Conclusions
These results indicate that differences in target lists predicted from different databases lead to differences in enrichment analysis, which in turn leads to differences in disease prediction coverage. The most important thing is to make sure that the characteristics of TCM bioinformatics databases match researchers’ own research objectives. Using a merged target list predicted by at least two TCM bioinformatics databases for analysis may provide more unbiased, complete, and wider range of results than using a single database without recognizing their characteristics.
Acknowledgments
We are grateful to MS Park for providing technical support. This work was supported by the Collection of Clinical Big Data and Construction of Service Platform for Developing Korean Medicine Doctor with Artificial intelligence research project [grant number: KSN1922110].
Data Availability Statement
The original contributions presented in the study (i.e. the total lists of ingredient, target, analysis results) are included in the article/Supplementary tables, further inquiries can be directed to the corresponding author.
References
-
Zhang YQ, Mao X, Guo QY, Lin N, Li S. Network pharmacology-based approaches capture essence of Chinese herbal medicines. Chin. Herb. Med. 2016;8(2):107-16.
[https://doi.org/10.1016/S1674-6384(16)60018-7]
-
Che CT, Wang ZJ, Chow M, Lam C. Herb-herb combination for therapeutic enhancement and advancement: theory, practice and future perspectives. Molecules. 2013;18(5):5125-41.
[https://doi.org/10.3390/molecules18055125]
-
Lim B. Korean medicine coverage in the National Health Insurance in Korea: present situation and critical issues. Integr. Med. Res. 2013;2(3):81-8.
[https://doi.org/10.1016/j.imr.2013.06.004]
-
Kwon S, Heo S, Kim D, Kang S, Woo JM. Changes in trust and the use of Korean medicine in South Korea: a comparison of surveys in 2011 and 2014. BMC complement. Altern. Med. 2017;17:1-10.
[https://doi.org/10.1186/s12906-017-1969-8]
-
Tanaka MM, Kendal JR, Laland KN. From Traditional Medicine to Witchcraft: Why Medical Treatments Are Not Always Efficacious. PloS One. 2009;4(4):5192.
[https://doi.org/10.1371/journal.pone.0005192]
-
Ernst E. Methodological aspects of traditional Chinese medicine (TCM). Ann.Acad. Med. Singap. 2006;35(11):773-4.
[https://doi.org/10.47102/annals-acadmedsg.V35N11p773]
-
Li B, Tao W, Zheng C, Shar PA, Fu Y, Wang Y. Systems pharmacology-based approach for dissecting the addition and subtraction theory of traditional Chinese medicine: an example using Xiao-Chaihu-Decoction and Da-Chaihu-Decoction. Comput. Biol. Med. 2014;53:19-29.
[https://doi.org/10.1016/j.compbiomed.2014.05.007]
-
Hopkins AL. Network pharmacology: the next paradigm in drug discovery. Nat. Chem. Biol. 2008; 4(11):682-90.
[https://doi.org/10.1038/nchembio.118]
-
Li S, Fan TP, Jia W, Lu A, Zhang W. Network pharmacology in traditional Chinese medicine. Evid. Based Complement. Alternat. Med. 2014:e138460.
[https://doi.org/10.1155/2014/138460]
-
Jiao X, Jin X, Ma Y, Yang Y, Li J, Liu R, et al. A comprehensive application: Molecular docking and network pharmacology for the prediction of bioactive constituents and elucidation of mechanisms of action in component-based Chinese medicine. Comput. Biol. Chem. 2021;90:107402.
[https://doi.org/10.1016/j.compbiolchem.2020.107402]
-
Pan Z, Li M, Jin Z, Sun D, Zhang D, Hu B, et al. Research status of Chinese medicine formula based on network pharmacology. Pharmacol. Res. -Mod. Chin. Med. 2022;4:100132.
[https://doi.org/10.1016/j.prmcm.2022.100132]
-
Ru J, Li P, Wang J, Zhou W, Li B, Huang C, et al. TCMSP: a database of systems pharmacology for drug discovery from herbal medicines. J. Cheminformatics. 2014; 6:1-6.
[https://doi.org/10.1186/1758-2946-6-13]
-
Liu Z, Guo F, Wang Y, Li C, Zhang X, Li H, et al. BATMAN-TCM: a bioinformatics analysis tool for molecular mechANism of traditional Chinese medicine. Sci. Rep. 2016;6(1):1-11.
[https://doi.org/10.1038/srep21146]
-
Huang L, Xie D, Yu Y, Liu H, Shi Y, Shi T, et al. TCMID 2.0: a comprehensive resource for TCM. Nucleic Acids Res. 2018;46(D1):D1117-20.
[https://doi.org/10.1093/nar/gkx1028]
-
Bi YH, Zhang L, Chen S, Ling Q. Antitumor mechanisms of curcumae rhizoma based on network pharmacology. Evid. Based Complement. Alternat. Med. 2018;2018(1):4509892.
[https://doi.org/10.1155/2018/4509892]
- Choi M, Yang W, Lee B, Cho S. Basic network pharmacological analysis of Salvia miltiorrhiza root for further application to an animal stroke model. Herb. Formula Sci. 2021;29(1):19-31.
-
Kim J, Lee KP, Kim MR, Kim BS, Moon BS, Shin CH, et al. A network pharmacology approach to explore the potential role of Panax ginseng on exercise performance. Phys. Act. Nutr. 2021;25(3):28-35.
[https://doi.org/10.20463/pan.2021.0018]
- Park S, Lee B, Jin M, Cho S. Comparison of network pharmacology based analysis on white ginseng and red ginseng. Herb. Formula Sci. 2020;28(3):243-54.
-
Zhang R, Zhu X, Bai H, Ning K. Network pharmacology databases for traditional Chinese medicine: review and assessment. Front. pharmacol. 2019;10,00123.
[https://doi.org/10.3389/fphar.2019.00123]
-
Liu Y, Ai N, Keys A, Fan X, Chen M. Network pharmacology for traditional Chinese medicine research: methodologies and applications. Chin. Herb. Med. 2015;7(1):18-26.
[https://doi.org/10.1016/S1674-6384(15)60015-6]
-
Guo J, Shang E, Zhao J, Fan X, Duan J, Qian D, et al. Data mining and frequency analysis for licorice as a “Two-Face” herb in Chinese Formulae based on Chinese Formulae Database. Phytomedicine. 2014;21(11):1281-6.
[https://doi.org/10.1016/j.phymed.2014.07.006]
- Joint Textbook Editing Committee from universities of Korean medicine. Materia medica. 2nd ed. South Korea: Younglimsa; 2011.
- Yu X, Huang Zh. Refined translation of Compendium of Materia Medica. 1st ed. China: Scientific and Technical Documents Publishing House; 1999.
-
Yu H, Chen J, Xu X, Li Y, Zhao H, Fang Y, et al. A systematic prediction of multiple drug-target interactions from chemical, genomic, and pharmacological data. PLoS One. 2013;7(5):37608.
[https://doi.org/10.1371/journal.pone.0037608]
-
Miao R, Meng Q, Wang C, Yuan W. Bibliometric Analysis of Network Pharmacology in Traditional Chinese Medicine. Evid. Based Complement. Alternat. Med. 2022;2022(1):1583773.
[https://doi.org/10.1155/2022/1583773]
-
Sabbadin C, Bordin L, Dona G, Manso J, Avruscio G, Armanini D. Licorice: From Pseudohyperaldosteronism to Therapeutic Uses. Front. Endocrinol. 2019;10:484.
[https://doi.org/10.3389/fendo.2019.00484]
-
Yoshino T, Shimada S, Homma M, Makino T, Mimura M, Watanabe K. Clinical Risk Factors of Licorice-Induced Pseudoaldosteronism Based on Glycyrrhizin-Metabolite Concentrations: A Narrative Review. Front. Nutr. 2021;8:719197.
[https://doi.org/10.3389/fnut.2021.719197]
-
Minnetti M, Alcubierre DD, Bonaventura I, Pofi R, Hasenmajer V, Tarsitano MG, et al. Effects of licorice on sex hormones and the reproductive system. Nutrition. 2022;103:111727.
[https://doi.org/10.1016/j.nut.2022.111727]
-
Boots AW, Haenen G, Bast A. Health effects of quercetin: From antioxidant to nutraceutical. Eur. J. Pharmacol. 2008;585(2-3):325-37.
[https://doi.org/10.1016/j.ejphar.2008.03.008]
-
Chen AY, Chen YC. A review of the dietary flavonoid, kaempferol on human health and cancer chemoprevention. Food Chem. 2013;138(4):2099-107.
[https://doi.org/10.1016/j.foodchem.2012.11.139]
-
Deutch MR, Grimm D, Wehland M, Infanger M, Kruger M. Bioactive Candy: Effects of Licorice on the Cardiovascular System. Foods. 2019;8(10):495.
[https://doi.org/10.3390/foods8100495]
-
Yang R, Wang LQ, Yuan BC, Liu Y. The Pharmacological Activities of Licorice. Planta Med. 2015;81(18):1654-69.
[https://doi.org/10.1055/s-0035-1557893]
-
Wahab S, Annadurai S, Abullais SS, Das G, Ahmad W, Ahmad F, et al. Glycyrrhiza glabra (Licorice): A Comprehensive Review on Its Phytochemistry, Biological Activities, Clinical Evidence and Toxicology. Plants. 2021;10(12):2751.
[https://doi.org/10.3390/plants10122751]