
생성형 거대언어모델의 의학 적용 현황과 방향 : 동아시아 의학을 중심으로
Ⓒ The Society of Pathology in Korean Medicine, The Physiological Society of Korean Medicine
Abstract
The rapid advancement of generative large language models has revolutionized various real-life domains, emphasizing the importance of exploring their applications in healthcare. This study aims to examine how generative large language models are implemented in the medical domain, with the specific objective of searching for the possibility and potential of integration between generative large language models and East Asian medicine. Through a comprehensive current state analysis, we identified limitations in the deployment of generative large language models within East Asian medicine and proposed directions for future research. Our findings highlight the essential need for accumulating and generating structured data to improve the capabilities of generative large language models in East Asian medicine. Additionally, we tackle the issue of hallucination and the necessity for a robust model evaluation framework. Despite these challenges, the application of generative large language models in East Asian medicine has demonstrated promising results. Techniques such as model augmentation, multimodal structures, and knowledge distillation have the potential to significantly enhance accuracy, efficiency, and accessibility. In conclusion, we expect generative large language models to play a pivotal role in facilitating precise diagnostics, personalized treatment in clinical fields, and fostering innovation in education and research within East Asian medicine.
Keywords:
Generative large language model, East Asian medicine서 론
인공지능의 발전과 함께 거대언어모델이 출현하면서 인간이 자연어를 통해 기계와 상호작용하고 이를 처리하는 능력이 크게 향상되었다1). 거대언어모델은 인공 신경망 구조에 기반을 두고 있으며, 기존 언어모델과의 차이에 대해서는 다양한 견해가 존재하나 대체로 막대한 수의 내부 파라미터와 학습 데이터를 특징으로 한다2). 2010년대 후반부터 급속하게 발전한 거대언어모델의 본격적인 시작은 2017년 트랜스포머(Transformer) 모델의 도입으로 여겨진다2)(Fig. 1). 트랜스포머 모델은 인코더-디코더의 순차적 구조를 가지며, 자연어 처리에서 오랜 난제였던 텍스트 내 장거리 의존성 문제*를 효과적으로 해결하기 위한 어텐션(Attention) 메커니즘을 도입하였다3). 이후 2018년에는 트랜스포머의 인코더 구조만을 이용한 BERT (Bidirectional Encoder Representations from Transformers)가 개발되었다. BERT는 언어 내의 문맥과 뉘앙스를 이해하고 표상해내는 능력에서 진일보하여 많은 관심을 받았다4). 최근에는 OpenAI의 GPT (Generative Pre-trained Transformer), Meta의 LLaMA (Large Language Model Meta AI) 등 트랜스포머의 디코더 구조를 이용한 생성형 거대언어모델들이 주목받고 있다5,6). 이들은 인터넷, 책, 심지어는 다른 생성형 거대언어모델이 생성한 텍스트 등의 방대한 데이터를 학습하여 언어의 구조, 뉘앙스 및 문맥을 학습한다2). 이러한 모델들은 인간 수준으로 논리적이고 문맥적으로 유관한 텍스트를 생성하는 능력을 입증하였으며, 의료서비스, 교육, 고객 응대 등 다양한 분야에서 활용되고 있다7-9). 이는 실생활의 형태를 바꿀 수 있는 생성형 거대언어모델의 광활한 잠재력을 보여준다. 하지만, 이러한 모델은 부정확하거나 오해의 소지가 있는 정보를 생성하는, 소위 '환각(hallucination)'의 가능성이 있으며, 학습 데이터에 존재하는 편향에 의존해 결과에 영향을 미칠 수 있는 등의 한계가 있다10). 이를 해결하기 위해 다양한 전이학습(Transfer learning) 및 파인튜닝(Fine-tuning) 방법론과 검색증강생성(Retrieval-augemented generation) 등의 모델증강 대안이 개발되었다. 전이학습과 파인튜닝은 유사한 개념으로, 추가적인 학습을 통해 특정 도메인이나 작업에 맞게 모델을 업데이트하거나 편향을 완화하여 다양한 도메인에서 신뢰성과 적용 가능성을 개선한다11). 검색증강생성은 언어모델의 생성 기능과 외부 지식 소스를 결합하여 응답의 정확도와 관련성을 높인다12).

Overview of Transformer-based language models: evolution, capabilities, challenges, and mitigation strategies.
의학의 관점에서 바라볼 때, 거대언어모델의 통합은 효율적이고 접근하기 쉬우면서도 개인 맞춤화된 의료서비스로의 전환을 의미한다. 그 활용은 대규모 의학 문헌 및 텍스트 데이터 분류 및 분석, 정보 검색 등의 초기 응용 작업에서 발전하여, 현재에 이르러서는 더욱 직접적인 환자 관리, 예를 들어 환자 진단, 환자 교육 등에까지 범위를 넓혔다13-17). 대화형 언어모델인 ChatGPT는 미국, 중국, 일본 등 다양한 국가의 의사 면허시험을 통과하였다. 이 같은 성과는 모델이 의학 분야에 대한 깊은 이해를 바탕으로 진단 및 교육 도구로서의 큰 잠재력을 가지고 있음을 보여주며, 모델 활용의 당위성을 확립하였다18-20). 기본적인 의학 지식을 넘어 거대언어모델은 복잡한 임상 상황 이해에 대한 역량을 보였으며, 치료 계획 제공과 의료 훈련 목적으로서의 환자 상호작용 시뮬레이션을 위해 이용된 경우도 존재하였다21,22). 이러한 발전은 의과학의 다면적 특성을 처리함에 있어 모델의 보편성을 드러내며, 동시에 기초 및 임상의학적 문맥에서 보건의료인의 기량을 더욱 상승시키는데 모델이 이용될 수 있음을 나타낸다.
최근 인공지능 기술을 이용한 동아시아 의학의 현대화 시도로서, 거대언어모델과 동아시아 의학 간의 융합 연구가 진행되고 있다. 동아시아 의학 문헌 텍스트 데이터를 이용하여 영역 특화 모델을 구축하거나 정확한 임상 진단을 유도하는 연구가 그 예이다23,24). 이러한 연구 결과는 생성형 언어모델은 개인 맞춤 및 전인적인 접근법을 강조하는 동아시아 의학의 내재적 특성을 이해하고 임상의들을 보조하는 데에 큰 도움이 될 수 있음을 시사한다. 하지만, 아직 초기 단계로서 모델의 잠재 가능성을 완전히 활용하기 위해서는 해결해야 할 한계점이 존재한다. 표준화, 공공화, 디지털화 되지 않은 데이터 양식이 대표적인 예이다25,26). 또한, 주류 모델들이 영어 텍스트를 중심으로 학습된 반면, 동아시아 의학계의 주요 데이터가 한문을 비롯한 동아시아 언어로 이루어진 것은 추가적인 어려움을 초래한다26).
동아시아 의학은 서양 의학과 다른 독특한 이론과 치료 체계를 가지고 있으며, 이러한 차이는 동아시아 의학에서의 거대언어모델 활용 시 고려되어야 할 중요한 요소이다. 따라서 본 연구는 동아시아 의학의 특수성과 거대언어모델의 융합 가능성을 탐색하고자 하는 목적에서 시작되었다. 본 연구는 생성형 거대언어모델의 서양의학 및 동아시아 의학에 대한 기존 연구 성과들에 대한 종합적인 현황 개요를 제공하며, 이를 기반으로 생성형 거대언어모델의 동아시아 의학 연구 적용에 대한 잠재 가능성과 향후 연구 방향성을 고찰한다. 연구 과정에서 동아시아 의학 데이터의 표준화, 디지털화 및 축적의 필요성 등의 도전 과제 또한 확인하였다. 이러한 한계점과 향후 연구방향은 동아시아 의학에서 거대언어모델을 실용적으로 활용하기 위한 통찰을 제공한다. 또한 보다 실용적인 관점에서 동아시아 의학의 기초연구 및 임상 환경을 개선한다.
연구대상 및 방법
1. 자료 선정 및 제외 기준
본 연구는 서양의학 및 동아시아 의학 분야에서 생성형 거대언어모델을 활용한 연구들을 수집하고, 자료 선정 및 제외 기준에 따라 선별하였다. 자료의 선정 및 제외 기준으로는 트랜스포머 모델 도입 이후의 연구, 서양의학 및 동아시아 의학 분야에서의 거대언어모델 활용에 관한 연구, 자연어 또는 인공어 생성이 주요하게 활용된 연구를 포함하고, BERT와 같이 트랜스포머 모델의 인코더 부분만 사용한 연구, 서양의학 연구 한정으로 특정 국가에 국한되어 연구 결과 일반화에 한계가 있는 연구는 제외하였다.
2. 검색 전략
검색식은 생성형 거대언어모델, 서양의학, 동아시아 의학에 관련된 문헌을 포괄적으로 조사하도록 아래와 같이 설계되었다.
서양의학 분야에서는 PubMed, Google Scholar 및 arXiv에서 "Medicine", "Medical", "Large Language Model", “Generative”, “Generation”, "GPT", "PaLM", "Clinical", "Healthcare", "Diagnosis" 등의 키워드를 조합하여 검색하였다.
동아시아 의학 분야에서는 PubMed, Google Scholar, CNKI에서 “Traditional medicine”, "Chinese medicine", "TCM", "Korean medicine", "TKM", "Kampo", "Japanese medicine", “Herbal medicine“, ”Acupuncture“, "Large Language Model", "GPT", “Generative”, “Generation” 등의 키워드를 사용하여 검색하였다. 추가적으로 한국 학술지 인용색인(KCI)에서 "한의학", “한의약”, “한약”, “침구의학”, “경혈”, "거대언어모델", "대규모 언어 모델", "생성형" 등의 키워드를 사용하여 검색하였다.
3. 연구 분류 및 분석
서양의학 및 동아시아 의학적 측면에서 생성형 거대언어모델의 기본 성능과 증강된 성능을 구분해서 보기 위해, 선별된 연구를 모델의 개발 방법에 따라 분류하였다(Fig. 2). 기본 모델로 진행된 연구(이하 기본모델 연구)와 추가 학습을 통해 성능이 향상된 모델에 관한 연구(이하 증강모델 연구), 크게 두 가지 카테고리로 분류하였다. 추가로, 증강모델 연구는 증강의 목적에 따라 두 가지 범주로 세분화하였다. 첫 번째는 범용성을 높이기 위한 모델 증강으로, 다양한 데이터셋과 작업에 적용할 수 있는 의학 및 동아시아 의학 특화 모델을 개발하는 것이 목적이다. 두 번째는 특정 사용 환경에 대한 특이성을 높이기 위한 모델 증강으로, 특정 작업이나 데이터셋에 최적화된 모델을 개발하는 것이 목적이다.
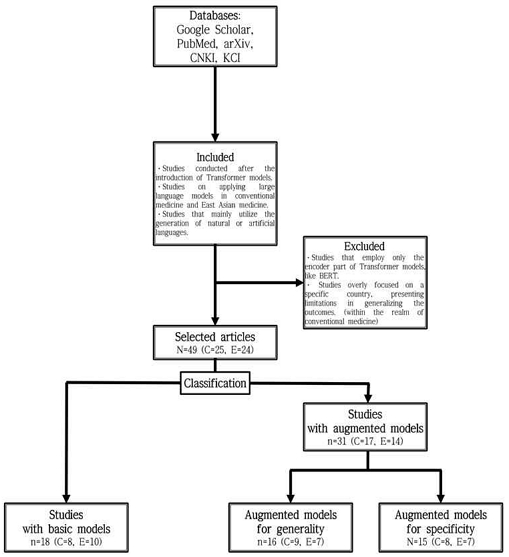
Flow chart of study selection and classification. C and E indicate conventional medicine and East Asian medicine, respectively.
각 연구의 분류, 목적 및 결과를 중심으로 내용을 정리하고, 서양의학 분야에서 생성형 거대언어모델의 적용 사례를 개괄적으로 조망하였다. 이를 기반으로, 우리는 생성형 거대언어모델이 동아시아 의학 분야에서 발휘할 수 있는 잠재력과 가능성을 탐색하고 향후 연구 방향성에 대한 시사점을 제시하였다.
결 과
1. 서양의학에서의 생성형 거대언어모델 적용 현황
Table 1에 서양의학 연구에 생성형 거대언어모델이 적용된 현황을 정리하였다. 25개 논문이 선별되었으며, 그 중 기본모델 연구 8개, 범용성 증강모델 연구 9개, 특수성 증강모델 연구 8개가 포함되었다.
기본모델 연구들에서는 GPT 기반 모델들을 중심으로, BARD, LLaMA2까지 포함된 사전학습 모델들이 의료 분야의 다양한 맥락에서 능력을 발휘한다는 것이 강조되었다. 이러한 모델들은 정보 추출, 질병 진단, 전정신경초종과 같은 특정 조건의 관리에 이르기까지 다양한 의료 맥락에서 고도의 정확도, 재현율, 속도를 보였다. 방사선 종양학과 류마티스학 분야에서는 인간 전문가를 능가하는 성능을 보여주기도 하였다. 특히 임상 텍스트로부터의 정보 추출은 zero-shot과 few-shot을 통해 좋은 성적을 거두었는데, 이는 추가적인 모델 파라미터의 튜닝 없이 단순히 적절한 프롬프트나 소수의 예시를 제공함으로써 이미 충분한 성능을 보인다는 점에서 주목할 만하다. 게다가, 임상 텍스트에서 개인 신상과 같은 민감한 정보의 비식별화에 잠재력을 드러냈다. 이는 기존에 의료 분야에서의 인공지능 모델 활용 시 중요한 난관 중 하나였던 개인정보보호 이슈에 대한 해결책을 제시할 수 있다는 점에서 의미가 있다. 이러한 연구 결과는 기본 모델들이 이미 충분한 수준의 의학 지식을 갖추고 있으며, 의료 연구, 진단, 정보 추출 등 다양한 분야에서 활용 가능함을 시사한다.
의학 분야에서의 범용성을 증강한 모델로는 Med-PaLM2, BiomedGPT, PMC-LLaMA, MEDITRON-70B, ChatDoctor, Almanac, MedEdit, MedAlpaca, CLINICALGPT등이 있으며, 이들은 다양한 의학 질의응답 작업에서 뛰어난 성능을 보였다. 이 모델들은 파인튜닝을 통해 MedQA, PathVQA, USMLE와 같은 특화된 데이터셋에서의 우수성을 입증하였을 뿐만 아니라, 검색증강생성에 기반하여 더 넓은 범위의 의학 지식 질문에 대해서도 탁월한 성능을 달성하였다. 특히, 이 증강모델들은 ChatGPT-4, BERT, LLaMA와 같은 이전 기본모델이 도달한 의학 영역에서의 벤치마크를 뛰어넘는 높은 정확도, 더 적은 파라미터로의 효율성, 적대적 상황에서의 안전성 개선을 달성하였다. 이러한 모델들은 의료 지식 추출 및 진단의 질과 신뢰성을 현저히 향상시킬 잠재력을 가지며, 의료 분야에서 AI 응용의 새로운 기준을 설정한다.
마지막으로, DRG-LLaMA, RadOnc-GPT, PhenoGPT, CLIPSyntel, Health-LLM, SkinGPT-4, AMIE, EHR-KnowGen 등은 진단명 기준 환자군 예측, 방사선 종양학, 표현형 정보 추출, 대화형 진단 및 멀티모달 진단, 질병 예측과 같은 의학 분야에서 특화된 작업에 대한 성능을 증강하고자 하였다. 특히 CLIPSyntel, Health-LLM, SkinGPT-4, EHR-KnowGen 모델은 검색증강생성 및 멀티모달 구조의 방법론을 채택하여 비교 모델들보다 우수한 진단 성능을 보여주며, 의료 진단 및 치료 계획의 정밀도와 효율성을 크게 향상시켰다. 이러한 연구 결과들은, 특정 의료 영역에 대한 정밀도와 적응력을 맞춤화한 증강모델들이 세부적이고 특화된 의학 진단 및 치료 방법의 개선에 큰 잠재력을 가지고 있음을 보여준다.
2. 동아시아 의학에서의 생성형 거대언어모델 적용 현황
Table 2에 생성형 거대언어모델이 동아시아 의학에 적용된 연구 사례를 정리하였다. 총 24개 논문이 포함되었으며, 이 중 기본 모델 연구가 10개, 범용성 증강모델 연구가 7개, 특수성 증강모델 연구가 7개였다. 개별 모델에서는 ChatGPT와 관련된 논문이 11개로 가장 많은 비중을 차지하였다.
Li 등52)은 ChatGPT-3.5 모델을 기반으로 중의학 지식의 평가를 진행하였다. 이 연구에서는 TCM-QA 데이터셋의 다양한 중의학 문제 유형에 따라 변동적인 성능을 보였으며, 참/거짓 형식에서 가장 높은 정밀도(0.688)를 달성했고, 객관식 시나리오에서는 가장 낮은 성능(0.241)을 보였다. 또한 중국어 프롬프트에서 영어보다 더 좋은 결과를 나타냈다. 이러한 결과는 추가적인 과정 없이 생성형 거대언어모델을 전문 중의학 지식에 적용할 때의 가능성과 한계를 모두 보여준다.
Hsu 등53)은 ChatGPT-3.5를 활용하여 약물 상담 응답에 대한 정확성과 적절성을 평가하였다. 전문가 평가에서, 모델은 일반 대중을 위한 약물 상담 응답에 대해 61%의 적절성을 달성하였다. 하지만, 아스피린과 8종 본초 간의 잠재적 상호작용을 묻는 질문에서는 50%의 응답이 부적절하다고 판단되었다. 이 결과는 이 연구는 거대언어모델이 의약학 분야에서는 우수한 성능을 보이나, 동아시아 의약학 분야에서는 추가적인 최적화와 지식 통합이 필요함을 암시한다.
Lee54)는 ChatGPT를 침구의학 교육의 보조 도구로서 평가하였는데, ChatGPT-4는 ChatGPT-3.5보다 더 많은 경혈 제안을 생성하는 능력이 뛰어났다(9.0±1.1 및 5.3±0.6). 이 연구는 ChatGPT가 침구의학의 학습을 촉진하고 비판적 사고를 자극하는 교육 도구로 유용할 수는 있지만, 기존의 진단 및 치료 방법을 대체할 수는 없다고 주장하였다.
Chen 등55)은 ChatGPT-4가 생성한 중의학 처방의 안전성과 적합성을 탐구하였다. 연구 결과는 ChatGPT-4가 생성한 처방과 인간 전문가가 구성한 처방 사이에 통계적으로 유의한 차이가 없다고 밝혔다. 더욱이, 튜링 테스트에서 모델 생성 처방의 약 51.11%가 인간이 만든 것으로 오판되었다. 이는 중의 처방 구성원리에 있어 모델의 뛰어난 모방 및 이해 능력을 보여준다고 해석된다.
Jang 등56)은 한의사 국가 면허시험을 통해 ChatGPT-4의 한의학적 지식과 이해도를 조사하였다. 연구진은 Chain of Thought 등의 프롬프트 엔지니어링 기법을 통해 정답률을 초기 51.82%로부터 66.18%까지 상승시키며, 한의사 국가시험 합격 기준을 충족시켰다. 이 결과는 모델이 한의사와 같은 기본적인 수준의 한의학 지식을 갖추고 있음을 나타내며, 앞으로 다양한 한의학 관련 작업에도 적용 가능함을 의미한다.
Kim 등57)은 ChatGPT-4가 한의학 치료에 대한 개요를 제공하는 예를 보이고, 임상의와 의료소비자가 개괄적인 임상 한의학 정보를 얻는 데 유용한 도구임을 제시하였다.
Liu 등58)은 다양한 생성형 거대언어모델(ChatGPT-3.5, ChatGPT-4, ChatGLM, LLaMA, Alpaca, Vicuna)의 의료 질문응답 능력을 평가하고 개선하는 데 CMExam이라는 벤치마크 데이터셋을 사용하였다. 중의학 및 중의약 문항에서 ChatGPT-4가 ChatGPT-3.5, ChatGLM, fine-tuned ChatGLM 대비 가장 높은 성능을 보였으며, 각각 정확도 53.5% 및 45.4%를 달성하였다.
Zhu 등59)은 ChatGPT-3.5/4, Claude-2, Gemini-pro, Ernie Bot, Qwen-max 등의 모델을 사용하여 중의학 지식에 대한 중국 및 서구권 언어모델의 비교 평가를 실시하였다. 이 연구에서는 중국에서 개발된 모델이 서구권 모델들을 크게 앞서는 것을 확인하였으며, 중국 모델은 평균 정확도 78.4%를, 서구 모델들은 35.9%를 기록하였다. 이 결과는 동아시아 의학과 같은 특정 문화적 맥락에서, 언어모델 훈련 시의 지역 언어 및 문화 데이터의 포함이 모델의 성능을 향상시키는데 중요함을 제시한다.
Zhang 등60)은 iFLYTEK Spark Cognitive Large Model을 기반으로 중의학 지식 그래프 구축을 목적으로 한 연구를 진행하였다. 이 모델은 특히 few-shot 학습 기법과 자체 검증 전략을 최적화할 때, ChatGPT와 비교하여 개체 및 관계 추출 작업에서 우수한 성능을 보였다. 이 연구는 중의학 지식의 조직화 및 추출 작업의 효율성을 증진함에 있어 생성형 언어모델의 활용 가능성을 보여준다.
Park 등61)은 ChatGPT를 비롯한 거대언어모델이 개인 맞춤형 학습 계획과 학습 자료 제공, 지식 통합 및 커리큘럼 개발, 학생 평가 자동화, 가상 환자 시뮬레이션 실습, 그리고 연구 활동 등 한의학 교육에서 다양한 방식으로 활용 가능함을 제안하였다.
이러한 기본모델 연구는 ChatGPT를 중심으로 한 생성형 거대언어모델의 기본적인 동아시아 의학 이해도를 확인하고, 약물 상담, 처방 생성 및 동아시아 의학 교육 개선에서의 정확성 및 유용성을 보였다. 이들은 동아시아 의학에 대한 모델의 상당한 잠재력을 보여주며, 의료서비스 및 의료 교육의 근본적인 변화를 시사한다.
Li 등62)은 ChatGPT-3.5를 사용하여 송대 의학 처방 및 사례 데이터의 식별 및 분석을 목적으로 하였다. 이 모델은 송대의 중의학 처방 및 임상례 데이터의 다양한 측면을 효과적으로 식별하고 분류하였으며, 효과적인 처방과 비효과적인 처방을 구별하는 데 높은 정확도를 보였다. 또한, 데이터마이닝 및 통계적 접근법을 통해 질병 단계와 치료 전략을 분석하는 데 도움을 주었으며, 특히 온성 본초가 담을 치료하는 데 주로 사용됨을 식별하였다. 이러한 사례는 역사적 의학 텍스트에서 얻은 통찰력을 제시하며 현대 동아시아 의학 연구 및 임상에 있어 실용적 도구로서의 역할을 제안한다.
Zhu 등63)은 LLaMA2 기반의 Zhongjing 모델을 개발하여 중의학 지식을 향상시키고자 하였다. 이 모델은 중의학 분야에서 전문화된 응답을 생성하는 데 있어 기존 모델인 Chinese-LLaMA2 대비 TCMEval 점수가 6.49만큼 향상되는 성과를 보였다. 이 결과는 맞춤형 언어모델이 동아시아 의학 분야에서 자동 응답의 질을 개선할 잠재력을 보여준다.
Zhang 등64)은 Chinese-LLaMA를 기반으로 한 Qibo 모델을 중의학 지식으로 학습시켰는데, 이 모델은 ChatGPT-3.5, Chinese-LLaMA, BenTsao, DoctorGLM, HuatuoGPT, 그리고 Zhongjing을 포함한 기타 모델들과 비교하여 중의학 분야에서 가장 우수한 성능을 보였다. 이 연구는 중의학에서 Qibo가 가진 가능성을 강조하며, 동아시아 의학에서 유용성과 정확성을 개선하기 위한 생성형 거대언어모델 도메인 특화 훈련의 중요성을 부각한다.
Kang 등65)은 ChatGPT-4를 발판으로 한의학 분야에 특화된 검색증강생성 기법을 도입하였다. 해당 연구에서는 기존 검색증강생성에서 한의학 분야 텍스트 임베딩 모델 사용이 최적이 아님을 보이고, 대안으로서 생성형 모델을 이용하는 새로운 기법(Prompt-RAG)을 제시하였다. 모델은 다양한 한의학 질문에 대하여, ChatGPT-3.5, ChatGPT-4 및 기존 임베딩 벡터 기반 검색증강생성 모델 대비 관련성과 정보성 항목에서 통계적으로 유의하게 높은 점수를 달성하였다. 이것은 생성형 거대언어모델로 하여금 한의학적으로 체계화된 응답을 생성할 방법을 모색하였다는 점에서 유의하다.
Yang 등66)은 중의학 분야의 범용 모델 구축을 목표로, BLOOM-7B 모델의 파인튜닝을 통해 개발한 TCM-GPT-7B를 소개하였다. 중의 진단 및 방제 지식 검증을 위한 데이터셋과 의료기록 기반 진단 작업 데이터셋으로 진행된 두 실험에서, 모델은 각각 정확도 29%, 26%로 기준 모델(BLOOM-7B)에 비해 17%, 12%의 성능 개선을 보였다. 이는 중의학 지식 쿼리와 임상 진단에 대한 모델의 향상된 응답 능력을 보여주며, 동아시아 의학 분야에 대한 거대언어모델 적용의 범용성을 확립한 예시가 된다.
Tan 등67)은 Baichuan-7B 모델을 사용하여 중의학 지식에 대한 질의응답(MedChatZH) 모델 개발 연구를 진행하였다. 이 모델은 실제 중의학 의료 시나리오에서 LLaMA-Med와 ChatGLM-Med를 유의하게 능가하는 성과를 보였다. 이는 중의학 관련 코퍼스에 기반한 모델 개발 및 파인튜닝을 통해 보다 정확하고 상황에 맞게 적절한 응답을 유도함과 동시에 이를 통한 의료 상담 및 교육 환경에서의 적용 가능성을 제안한다.
Yang 등68)은 중의학 코퍼스 준비, 지식 표상, 지시사항 파인튜닝, 강화학습 등을 통한 지능형 중의학 진단 및 치료 연구의 강화와 혁신을 제시하였다.
위의 연구들은 동아시아 의학 관련 질의응답에서 관련성 및 정보성을 개선할 뿐만 아니라, 중의학 지식 적용, 진단 처방 작업 등에서도 상당한 진전을 보였다. 이들 모델은 검색증강생성과 파인튜닝을 통해 기본 생성형 거대언어모델의 범용성을 증강하는 방법과 더불어 동아시아 의학 적용에서의 질과 정확도를 유의하게 상승시킨 모범을 제시한다.
Pu 등69)은 파인튜닝된 RoBERTa와 UniLM을 기반으로 중의학 처방 생성을 위한 지식 증강 모델 (RoKEPG)을 구축하였다. 이 모델은 한의학 처방 생성 작업에서 GPT-2, BART, T5 등의 기준 모델들보다 더 높은 F1 점수를 달성하며, 중의학 처방 생성 분야에서 지식 증강 모델의 잠재력을 보여주었다.
Wang 등70)은 BART 모델을 활용한 개인 맞춤형 중의 처방 추천 및 임상 의사결정 지원 모델 개발에 초점을 맞추었다. 임상 증상 설명을 처방 텍스트로 전환하는 작업에서, 모델 실험은 상위 선택 5개, 10개, 15개 본초에 대해 각각 58.60%, 53.79%, 49.67%의 정확도를 달성하였다. 이러한 결과는 BILSTM, CPT, GPT-2 등의 기준 모델 정확도를 능가한 것으로, 개인의 임상적 증상에 기반한 맞춤형 중의 처방 추천을 제공함에 있어 BART 기반 학습 모델의 효율성을 입증하였다.
Li 등71)은 파인튜닝된 ChatGPT-3.5를 기반으로 경혈 위치의 관계 추출을 목적으로 한 연구를 수행하였는데, 이 모델은 BioBERT, LSTM 및 기본 ChatGPT-3.5, ChatGPT-4 등 다른 모델들과 비교하여 모든 측정 항목(정밀도, 재현율, F1 점수)에서 우수한 성능을 보였다. 이 결과는 생성형 거대언어모델에 기반하여 동아시아 의학 내의 세부 분야에서 지식 추출 작업의 정확성과 효율성을 향상시킬 수 있는 가능성을 보인다.
Zhou 등72)은 ChatGLM 모델에 기반하여, 중의학적 유행병 상황에 특화된 대화형 모델 (EpidemicCHAT)을 개발하였다. 모델은 중의 유행병 처방 생성 작업에서 BLEU-2, BLEU-3, BLEU-4, ROUGE-L, METEOR 등의 메트릭에 걸쳐 Chinese-alpaca-plus, ChatGLM, ChatGPT 모델에 비해 가장 높은 성적을 보였다. 이 성과는 중의학적 유행병에 초점을 맞추어 적절한 중의 처방을 생성하는 과정에서 파인튜닝 기법과 이를 활용하여 구축된 EpidemicCHAT의 역량을 강조한다.
Liu 등73)은 ChatGLM-6B를 기반으로 한 CPMI-ChatGLM 모델을 사용하여 중국 특허 의약품 지침을 생성하는 연구를 진행하였다. 이 모델은 BLEU, ROUGE, BARTScore 지표에서 4개의 LLM(Chinese-LLaMA-7B, Chinese-Alpaca-7B, Qwen-7B, Baichuan-7B)을 능가했으며, 인간 평가에서 최고의 SUS(Safety, Usability, Smoothness) 점수를 기록하였다. 이 결과는 동아시아 의학에서 유래한 의약품을 현대화하고 국제화하는 데 있어 생성형 거대언어모델의 실용적인 응용 가치를 나타낸다.
Wang 등74)은 ChatGLM과 ChatGLM-2를 기반 모델로써 중의학 방제 분류를 위한 보조 모델 개발을 진행하였다. 파인튜닝 기법이 적용된 ChatGLM2-6b와 ChatGLM-6b 모델의 방제 분류 작업 정확도는 각각 71%, 70%로, ChatGLM-130b, ChatGPT, InternLM-20b 모델보다 우수한 성능을 보였다. 이 연구는 중의 방제 분류 작업에서의 파인튜닝을 통한 거대언어모델의 탁월한 역량을 보이며, 중의학 지식 내의 모호한 분류 체계을 명확하고 접근하기 쉬운 형태로 전환하는 가능성을 보여준다.
Zhang 등75)은 Ziya-LLaMA-13B-V1에 기반하여 중의학 원전 특화 대화형 모델(Huang-Di)을 구축하고자 하였다. 이 모델은 전반적인 중의학 지식을 평가하기 위해 원전으로부터 출제된 질의응답에서 通义千问, ShenNong-TCM, TCMLLM, ChatGPT-4 등의 대화형 거대언어모델 및 중의학 특화 모델보다 높은 전문가 평가 점수를 얻었다. 예방양생 분야에서 ChatGPT-4보다 근소하게 낮은 점수를 받았지만, 전반적으로 우수한 성능은 중의학 원전을 이해하고 분석하는 모델의 뛰어난 역량을 나타낸다.
이러한 특수성 증강모델의 적용은 동아시아 의학 원전에 대한 깊은 이해도에서부터 맞춤형 처방 생성까지 기초연구 및 임상의사결정에의 활용가능성을 입증한다. 이는 향후 생성형 거대언어모델이 동아시아 의학의 적용에 있어 다양한 작업적 특수 환경에 적응하여 세밀하게 교정될 수 있으며, 이에 따라 모델 내에서 지식이 어떻게 생성, 분류, 적용되는지에 대한 인간의 이해와 연구를 위한 새로운 방법을 제공한다는 점을 시사한다.
고 찰
1. 서양 및 동아시아 의학의 생성형 거대언어모델 적용 현황 비교
본 연구는 동아시아 의학에서의 생성형 거대언어모델의 활용방안에 대한 잠재 가치와 미래 방향을 제안하기 위해, 서양의학과 응용 가능성과 한계 측면에 집중하여 비교하고자 하였다. 연구 결과에 따르면, 서양의학은 생성형 거대언어모델의 지식 이해도와 적용 범위 측면에서 동아시아 의학보다 상대적으로 우위에 있었다. 이는 동아시아 의학에 대한 모델의 학습 데이터가 상대적으로 부족하기 때문으로 파악되며, 이를 극복하기 위해서는 추후 모델 증강을 위한 학습 데이터베이스의 구축이 중요하다는 것을 시사한다. 이를 위해, Kartchner 등30)의 연구와 같이 의무 기록, 논문과 같은 정형화된 텍스트에서의 정보 추출 및 임상에서의 환자 및 질환 관리 등의 작업에서 ChatGPT-4와 같은 기본모델을 활용할 수 있다. 이에 더해, Kang 등65)의 연구는 동아시아 의학 분야의 지식을 생성형 거대언어모델의 구조화된 응답으로 표현할 수 있는 방법을 고안하였다. 특히 Yang 등66)은 모델 학습 데이터를 확보하고 비교적으로 부족한 동아시아 의학 지식을 파인튜닝 기법을 통해 모델에게 보충한 예시를 보였다. 이는 동아시아 의학에게 서양의학 연구에서 폭넓게 시도되었던 범용성 및 특수성 증강 작업에 대한 가능성을 열어주며, 모델의 전반적인 동아시아 의학 지식의 증진과 더불어 다양한 체계 및 학파에 따른 진단 및 치료 특화 모델 생성으로 이어질 수 있다.
결과적으로, 이들 방법은 생성형 거대언어모델이 의료 분야에서 정확하고 표준적으로 응답하고 문서화하도록 돕는다. 동시에, 다른 새로운 모델의 학습에 필요한 데이터 제공자로서의 역할을 통해 선순환을 구축하는 잠재력을 가진다. 이는 향후 동아시아 의학 분야에서 거대언어모델의 전반적인 성능을 상향 평준화하며, 그 적용 범위를 확장한다.
2. 의료 분야에서의 생성형 거대언어모델의 한계와 해결책
생성형 거대언어모델의 사용은 환각(hallucination)이라는 본질적인 한계를 가지고 있다. 특히 정확성과 안전성이 중요시되는 의료 분야에서 환각 현상의 해결은 필수적이다. 환각의 완화를 위해 다양한 지도학습 데이터셋을 구축하거나 검색증강생성 모델을 사용하는 등의 전략이 제안되었지만, 부가적인 시간적, 경제적 비용 없이 환각을 해결하는 명확한 방법은 아직 발견되지 않았다76).
더불어, 의료 분야에서 모델 성능을 객관적으로 평가할 수 있는 자동화된 평가 시스템의 부재는 또 다른 도전 과제로 남아 있다. 본 연구에서 분석된 49개 논문 중 적어도 11개(약 22%)가 모델의 응답에 대해 인간 및 전문가 평가를 활용하였으며, 이는 모델 평가가 상당 부분 주관적 설문에 의존함을 의미한다. 이러한 문제를 해결하기 위해, 신뢰성 높은 자동화 모델 평가 프레임워크의 개발이 시급히 요구된다. Wang 등36)의 연구에서는 두 모델 간의 응답 품질을 ChatGPT-4에게 ‘Win’, ‘Tie’, ‘Lose’로 판단시키는 방식을 제안했는데, 이는 동아시아 의학 분야에서의 모델 평가에도 구현될 수 있는 방법으로 보인다.
한편, 환자 데이터를 활용한 의학 특화 모델의 학습은 환자의 개인정보 보호와 관련한 논란을 일으킬 수 있다. 모델 학습에 사용될 데이터의 범위 설정은 모델의 진단 정확성에 결정적인 영향을 미친다. Liu 등34)의 연구는 ChatGPT-4가 의료 텍스트를 비식별화하고 익명화하는 능력을 소개하였으며, 이는 개인정보 보호 문제에 대한 해결책으로 역할하리라 기대된다.
3. 생성형 거대언어모델 적용의 미래: 동아시아 의학의 관점에서
현재 우리는 기존 구현된 모델을 활용해 동아시아 의학 연구와 임상에 적용하기 시작하였으며, 동시에 향후 더욱 발전된 모델 학습을 위한 데이터를 축적하는 단계에 있다. 이 과정에서 기존 모델을 활용하여 표준화, 공공화, 디지털화된 데이터를 생산함으로써 미래의 더 발전된 언어모델 학습을 위한 기초 데이터로서 사용할 수 있다. 하지만 분야의 특성상 개인 수준 역량에는 한계가 존재하며, 보다 상위 차원에서의 대규모 동아시아 의학 데이터 공공화, 디지털화 작업의 병행이 필요하다. 이는 원전 데이터, 학술 데이터, 임상 데이터 등을 포함하며, 이러한 과정에서 이론체계와 변증 등의 서양의학과 구별되는 동아시아 의학의 특이성을 고려한 데이터 생성 및 취급 또한 요구된다. 비록 동아시아 언어를 통한 현시점 출시된 모델과의 상호작용이 영어에 비해 미숙하지만, Zhu 등59)의 예와 같이 향후 모델 규모 및 동아시아 언어 학습 데이터의 확장이 예상됨에 따라, 이러한 생성형 거대언어모델의 언어적 친숙성 및 성능은 자연스럽게 개선될 것이라 예상된다.
다양한 형태의 정보를 조합하여 활용하는 의료서비스의 기본적인 특성과 서양의학 분야에서의 생성형 거대언어모델 적용 현황을 고려할 때, 멀티모달 구조를 차용한 모델 개발이 동아시아 의학에서도 향후 핵심 전략으로 자리 잡을 것으로 보인다. 멀티모달 거대언어모델은 의료 이미지, 텍스트, 생체 데이터 등 다양한 데이터 유형을 처리하여 인간의 진단 과정을 모방할 수 있다77). 이는 동아시아 의학의 망문문절(望聞問切) 진단 방식과도 부합하여 임상 상황에서의 활용 가능성도 높다.
이와 함께, 지식증류(knowledge distillation) 기술의 사용은 의학 및 동아시아 의학 분야에서의 모델 발전에 기여할 것으로 예상된다. 지식증류 기술은 크고 복잡한 모델로부터 핵심 정보를 추출하여 성능은 최대로 유지한 채 더 작고 효율적인 모델을 생성하는 방법으로, 모델의 학습 효율성과 배포 용이성을 개선한다78). 지식 증류 기술을 활용하여, 의학 및 동아시아 의학 분야에서 큰 모델의 복잡한 지식과 패턴을 보다 간소화된 형태로 전달할 수 있다. 이는 모델의 크기와 복잡성을 줄이면서도 중요한 의료 지식을 유지하여, 실제 연구 및 의료 환경에서의 정보 접근성을 향상시킨다.
생성형 거대언어모델의 적용을 통해 교육 및 연구 분야에서도 혁신을 촉진할 수 있다. 이는 교수법 개선, 의료인 훈련 강화, 새로운 연구 가설의 제시와 검증 등 다양한 영역에서의 진보를 의미한다61,79). 결론적으로, 생성형 거대언어모델의 의학 분야 적용은 높은 잠재력을 가지고 이미 기대 이상의 성과를 내고 있으며, 동아시아 의학의 발전에도 기여할 중요한 기회를 제공한다(Fig. 3). 향후 연구와 기술 발전을 통해 현재 모델의 한계를 극복하고, 의료 분야에서의 활용성을 극대화하기를 기대한다.
결 론
본 연구는 의료 분야, 특히 동아시아 의학에서 생성형 거대언어모델의 현재 위치, 한계점, 그리고 가능성에 대해 탐구하였다. 서양의학과의 비교를 통해, 동아시아 의학에서 생성형 거대언어모델의 상대적인 부족함과 이를 개선하기 위한 데이터 축적의 중요성이 조명되었다. 더 나아가, 멀티모달 구조와 지식증류 기술 등의 접근 방법들이 제시되었으며, 이러한 기술적 발전은 동아시아 의학의 이해와 활용을 증진할 기회를 제공한다. 우리의 연구 결과는 생성형 거대언어모델이 의료 교육, 연구, 그리고 임상 실무 등 의학 분야 전반에서의 혁신을 가져올 수 있는 잠재력이 있음을 강조한다. 모델 활용성을 극대화함으로써, 정확한 진단, 개인 맞춤화된 치료, 그리고 효율적인 의료 자원 사용을 유도할 수 있다. 향후 추가 연구와 기술 발전을 통해 모델들을 개발 및 개선하고, 동아시아 의학 분야에서의 활용성을 높이는 방향으로 나아가야 할 것이다.
Acknowledgments
이 논문은 2022년도 가천대학교 교내연구비 지원에 의한 결과임.(GCU-202206720001)
Notes
References
-
Min B, Ross H, Sulem E, Veyseh APB, Nguyen TH, Sainz O, et al. Recent Advances in Natural Language Processing via Large Pre-trained Language Models: A Survey. ACM Comput Surv. 2023;56(2):Article 30.
[https://doi.org/10.1145/3605943]
- Minaee S, Mikolov T, Nikzad N, Chenaghlu M, Socher R, Amatriain X, et al. Large Language Models: A Survey. arXiv preprint arXiv:2402.06196, . 2024.
- Vaswani A, Shazeer N, Parmar N, Uszkoreit J, Jones L, Gomez AN, et al. Attention is all you need. Advances in neural information processing systems. 2017;30.
- Devlin J, Chang M-W, Lee K, Toutanova K. Bert: Pre-training of deep bidirectional transformers for language understanding. arXiv preprint arXiv:181004805, . 2018.
-
Fan L, Li L, Ma Z, Lee S, Yu H, Hemphill L. A bibliometric review of large language models research from 2017 to 2023. arXiv preprint arXiv:230402020, . 2023.
[https://doi.org/10.1145/3664930]
-
Gómez Cano CA, Sánchez Castillo V, Clavijo Gallego TA. Unveiling the Thematic Landscape of Generative Pre-trained Transformer (GPT) Through Bibliometric Analysis. Metaverse Basic and Applied Research. 2023;2:33.
[https://doi.org/10.56294/mr202333]
-
Li J, Dada A, Puladi B, Kleesiek J, Egger J. ChatGPT in healthcare: A taxonomy and systematic review. Computer Methods and Programs in Biomedicine. 2024;245:108013.
[https://doi.org/10.1016/j.cmpb.2024.108013]
-
Kasneci E, Sessler K, Küchemann S, Bannert M, Dementieva D, Fischer F, et al. ChatGPT for good? On opportunities and challenges of large language models for education. Learning and Individual Differences. 2023;103:102274.
[https://doi.org/10.1016/j.lindif.2023.102274]
-
Brynjolfsson E, Li D, Raymond LR. Generative AI at work. National Bureau of Economic Research; 2023.
[https://doi.org/10.3386/w31161]
- Huang L, Yu W, Ma W, Zhong W, Feng Z, Wang H, et al. A survey on hallucination in large language models: Principles, taxonomy, challenges, and open questions. arXiv preprint arXiv:231105232, . 2023.
-
Guo Y, Shi H, Kumar A, Grauman K, Rosing T, Feris R, editors. Spottune: transfer learning through adaptive fine-tuning. Proceedings of the IEEE/CVF conference on computer vision and pattern recognition; 2019.
[https://doi.org/10.1109/CVPR.2019.00494]
- Lewis P, Perez E, Piktus A, Petroni F, Karpukhin V, Goyal N, et al. Retrieval-augmented generation for knowledge-intensive nlp tasks. Advances in Neural Information Processing Systems. 2020;33:9459-74.
-
Lee J, Yoon W, Kim S, Kim D, Kim S, So CH, et al. BioBERT: a pre-trained biomedical language representation model for biomedical text mining. Bioinformatics. 2019;36(4):1234-40.
[https://doi.org/10.1093/bioinformatics/btz682]
-
Roy A, Pan S, editors. Incorporating medical knowledge in BERT for clinical relation extraction. Proceedings of the 2021 conference on empirical methods in natural language processing; 2021.
[https://doi.org/10.18653/v1/2021.emnlp-main.435]
-
Chen Y-P, Lo Y-H, Lai F, Huang C-H. Disease Concept-Embedding Based on the Self-Supervised Method for Medical Information Extraction from Electronic Health Records and Disease Retrieval: Algorithm Development and Validation Study. J Med Internet Res. 2021;23(1):e25113.
[https://doi.org/10.2196/25113]
-
Kottlors J, Bratke G, Rauen P, Kabbasch C, Persigehl T, Schlamann M, et al. Feasibility of Differential Diagnosis Based on Imaging Patterns Using a Large Language Model. Radiology. 2023;308(1):e231167.
[https://doi.org/10.1148/radiol.231167]
-
Kuckelman IJ, Yi PH, Bui M, Onuh I, Anderson JA, Ross AB. Assessing AI-Powered Patient Education: A Case Study in Radiology. Academic Radiology. 2024;31(1):338-42.
[https://doi.org/10.1016/j.acra.2023.08.020]
-
Mbakwe AB, Lourentzou I, Celi LA, Mechanic OJ, Dagan A. ChatGPT passing USMLE shines a spotlight on the flaws of medical education. Public Library of Science San Francisco, CA USA; 2023. p. e0000205.
[https://doi.org/10.1371/journal.pdig.0000205]
-
Wang H, Wu W, Dou Z, He L, Yang L. Performance and exploration of ChatGPT in medical examination, records and education in Chinese: Pave the way for medical AI. International Journal of Medical Informatics. 2023;177:105173.
[https://doi.org/10.1016/j.ijmedinf.2023.105173]
- Kasai J, Kasai Y, Sakaguchi K, Yamada Y, Radev D. Evaluating gpt-4 and chatgpt on japanese medical licensing examinations. arXiv preprint arXiv:230318027, . 2023.
-
Ferdush J, Begum M, Hossain ST. ChatGPT and Clinical Decision Support: Scope, Application, and Limitations. Annals of Biomedical Engineering. 2023.
[https://doi.org/10.1007/s10439-023-03329-4]
-
Holderried F, Stegemann–Philipps C, Herschbach L, Moldt J-A, Nevins A, Griewatz J, et al. A Generative Pretrained Transformer (GPT)–Powered Chatbot as a Simulated Patient to Practice History Taking: Prospective, Mixed Methods Study. JMIR Medical Education. 2024;10(1):e53961.
[https://doi.org/10.2196/53961]
-
Zhang M, Yang Z, Liu C, Fang L, editors. Traditional Chinese medicine knowledge service based on semi-supervised BERT-BiLSTM-CRF model. 2020 International Conference on Service Science (ICSS); 2020: IEEE.
[https://doi.org/10.1109/ICSS50103.2020.00018]
- Mucheng R, Heyan H, Yuxiang Z, Qianwen C, Yuan B, Yang G, editors. TCM-SD: A Benchmark for Probing Syndrome Differentiation via Natural Language Processing2022 October; Nanchang, China: Chinese Information Processing Society of China.
- Oh J. A Strategy for Disassembling the Traditional East Asian Medicine Herbal Formulas With Machine Learning. Journal of Oriental Medical Classics. 2023;36(2):23-34.
- Oh J. Comparison of Word Extraction Methods Based on Unsupervised Learning for Analyzing East Asian Traditional Medicine Texts. Journal of Oriental Medical Classics. 2019;32(3):47-57.
-
Agrawal M, Hegselmann S, Lang H, Kim Y, Sontag D. Large language models are zero-shot clinical information extractors. arXiv preprint arXiv:220512689, . 2022.
[https://doi.org/10.18653/v1/2022.emnlp-main.130]
- Guo E, Gupta M, Sinha S, Rössler K, Tatagiba M, Akagami R, et al. neuroGPT-X: toward a clinic-ready large language model. Journal of Neurosurgery. 2023:1-13.
-
Sivarajkumar S, Kelley M, Samolyk-Mazzanti A, Visweswaran S, Wang Y. An empirical evaluation of prompting strategies for large language models in zero-shot clinical natural language processing. arXiv preprint arXiv:230908008, . 2023.
[https://doi.org/10.2196/preprints.55318]
-
Kartchner D, Ramalingam S, Al-Hussaini I, Kronick O, Mitchell C, editors. Zero-Shot Information Extraction for Clinical Meta-Analysis using Large Language Models2023 July; Toronto, Canada: Association for Computational Linguistics.
[https://doi.org/10.18653/v1/2023.bionlp-1.37]
-
Zhang J, Sun K, Jagadeesh A, Ghahfarokhi M, Gupta D, Gupta A, et al. The Potential and Pitfalls of using a Large Language Model such as ChatGPT or GPT-4 as a Clinical Assistant. arXiv preprint arXiv:230708152, . 2023.
[https://doi.org/10.1093/jamia/ocae184]
-
Holmes J, Liu Z, Zhang L, Ding Y, Sio TT, McGee LA, et al. Evaluating large language models on a highly-specialized topic, radiation oncology physics. arXiv preprint arXiv:230401938, . 2023.
[https://doi.org/10.3389/fonc.2023.1219326]
-
Krusche M, Callhoff J, Knitza J, Ruffer N. Diagnostic accuracy of a large language model in rheumatology: comparison of physician and ChatGPT-4. Rheumatology International. 2024;44(2):303-6.
[https://doi.org/10.1007/s00296-023-05464-6]
- Liu, Zhengliang, et al. "Deid-gpt: Zero-shot medical text de-identification by gpt-4." arXiv preprint arXiv:2303.11032, (2023).
- Zhang K, Yu J, Yan Z, Liu Y, Adhikarla E, Fu S, et al. BiomedGPT: A Unified and Generalist Biomedical Generative Pre-trained Transformer for Vision, Language, and Multimodal Tasks. arXiv preprint arXiv:230517100, . 2023.
- Wang G, Yang G, Du Z, Fan L, Li X. ClinicalGPT: Large Language Models Finetuned with Diverse Medical Data and Comprehensive Evaluation. arXiv preprint arXiv:230609968, . 2023.
- Yunxiang L, Zihan L, Kai Z, Ruilong D, You Z. Chatdoctor: A medical chat model fine-tuned on llama model using medical domain knowledge. arXiv preprint arXiv:230314070, . 2023.
- Chen Z, Cano AH, Romanou A, Bonnet A, Matoba K, Salvi F, et al. Meditron-70b: Scaling medical pretraining for large language models. arXiv preprint arXiv:231116079, . 2023.
- Wu C, Zhang X, Zhang Y, Wang Y, Xie W. Pmc-llama: Further finetuning llama on medical papers. arXiv preprint arXiv:230414454, . 2023.
-
Zakka C, Shad R, Chaurasia A, Dalal AR, Kim JL, Moor M, et al. Almanac - Retrieval-Augmented Language Models for Clinical Medicine. NEJM AI. 2024;1(2):AIoa2300068.
[https://doi.org/10.1056/AIoa2300068]
-
Singhal K, Azizi S, Tu T, Mahdavi SS, Wei J, Chung HW, et al. Large language models encode clinical knowledge. Nature. 2023;620(7972):172-80.
[https://doi.org/10.1038/s41586-023-06291-2]
- Han T, Adams LC, Papaioannou J-M, Grundmann P, Oberhauser T, Löser A, et al. MedAlpaca--An Open-Source Collection of Medical Conversational AI Models and Training Data. arXiv preprint arXiv:230408247, . 2023.
- Shi, Yucheng, et al. "Mededit: Model editing for medical question answering with external knowledge bases." arXiv preprint arXiv:2309.16035, (2023).
-
Niu S, Ma J, Bai L, Wang Z, Guo L, Yang X. EHR-KnowGen: Knowledge-enhanced multimodal learning for disease diagnosis generation. Information Fusion. 2024;102:102069.
[https://doi.org/10.1016/j.inffus.2023.102069]
-
Yang J, Liu C, Deng W, Wu D, Weng C, Zhou Y, Wang K. Enhancing phenotype recognition in clinical notes using large language models: PhenoBCBERT and PhenoGPT. Patterns. 2024;5(1):100887.
[https://doi.org/10.1016/j.patter.2023.100887]
-
Ghosh A, Acharya A, Jain R, Saha S, Chadha A, Sinha S. Clipsyntel: Clip and llm synergy for multimodal question summarization in healthcare. arXiv preprint arXiv:231211541, . 2023.
[https://doi.org/10.1609/aaai.v38i20.30206]
-
Wang H, Gao C, Dantona C, Hull B, Sun J. DRG-LLaMA : tuning LLaMA model to predict diagnosis-related group for hospitalized patients. npj Digital Medicine. 2024;7(1):16.
[https://doi.org/10.1038/s41746-023-00989-3]
- Jin M, Yu Q, Zhang C, Shu D, Zhu S, Du M, et al. Health-LLM: Personalized Retrieval-Augmented Disease Prediction Model. arXiv preprint arXiv:240200746, . 2024.
-
Zhou J, He X, Sun L, Xu J, Chen X, Chu Y, et al. Pre-trained Multimodal Large Language Model Enhances Dermatological Diagnosis using SkinGPT-4. medRxiv. 2023:2023.06.10.23291127.
[https://doi.org/10.1101/2023.06.10.23291127]
- Tu T, Palepu A, Schaekermann M, Saab K, Freyberg J, Tanno R, et al. Towards conversational diagnostic ai. arXiv preprint arXiv:240105654, . 2024.
- Liu Z, Wang P, Li Y, Holmes J, Shu P, Zhang L, et al. Radonc-gpt: A large language model for radiation oncology. arXiv preprint arXiv:230910160, . 2023.
- Yizhen L, Shaohan H, Jiaxing Q, Lei Q, Dongran H, Zhongzhi L. Exploring the Comprehension of ChatGPT in Traditional Chinese Medicine Knowledge. arXiv preprint arXiv:240309164, . 2024.
-
Hsu H-Y, Hsu K-C, Hou S-Y, Wu C-L, Hsieh Y-W, Cheng Y-D. Examining Real-World Medication Consultations and Drug-Herb Interactions: ChatGPT Performance Evaluation. JMIR Med Educ. 2023;9:e48433.
[https://doi.org/10.2196/48433]
-
Lee H. Using ChatGPT as a Learning Tool in Acupuncture Education: Comparative Study. JMIR Med Educ. 2023;9:e47427.
[https://doi.org/10.2196/47427]
- Chen Q, Ni J, Xu J, Gao X, Xia L. Generation of traditional Chinese medicine prescription driven by generative artificial intelligence GPT-4. China Pharmacy. 2023;34(23):2825-8.
-
Jang D, Yun T-R, Lee C-Y, Kwon Y-K, Kim C-E. GPT-4 can pass the Korean National Licensing Examination for Korean Medicine Doctors. PLOS Digital Health. 2023;2(12):e0000416.
[https://doi.org/10.1371/journal.pdig.0000416]
-
Kim T-H, Kang JW, Lee MS. AI Chat bot - ChatGPT-4: A new opportunity and challenges in complementary and alternative medicine. Integrative Medicine Research. 2023;12(3):100977.
[https://doi.org/10.1016/j.imr.2023.100977]
- Liu J, Zhou P, Hua Y, Chong D, Tian Z, Liu A, et al. Benchmarking Large Language Models on CMExam-A Comprehensive Chinese Medical Exam Dataset. Advances in Neural Information Processing Systems. 2024;36.
-
Zhu L, Mou W, Lai Y, Lin J, Luo P. Language and cultural bias in AI: comparing the performance of large language models developed in different countries on Traditional Chinese Medicine highlights the need for localized models. Journal of Translational Medicine. 2024;22(1):319.
[https://doi.org/10.1186/s12967-024-05128-4]
-
Zhang Y, Hao Y. Traditional Chinese Medicine Knowledge Graph Construction Based on Large Language Models. Electronics. 2024;13(7):1395.
[https://doi.org/10.3390/electronics13071395]
-
Park S-Y, Kim C-E. Enhancing Korean Medicine Education with Large Language Models: Focusing on the Development of Educational Artificial Intelligence. Journal of Physiology & Pathology in Korean Medicine. 2023;37(5):134-8.
[https://doi.org/10.15188/kjopp.2023.10.37.5.134]
-
Li M, Zheng X. Identification of Ancient Chinese Medical Prescriptions and Case Data Analysis Under Artificial Intelligence GPT Algorithm: A Case Study of Song Dynasty Medical Literature. IEEE Access. 2023;11:131453-64.
[https://doi.org/10.1109/ACCESS.2023.3330212]
-
Zhu J, Gong Q, Zhou C, Luan H. ZhongJing: A Locally Deployed Large Language Model for Traditional Chinese Medicine and Corresponding Evaluation Methodology: A Large Language Model for data fine-tuning in the field of Traditional Chinese Medicine, and a new evaluation method called TCMEval are proposed. Proceedings of the 2023 4th International Symposium on Artificial Intelligence for Medicine Science. 2024;1036-42.
[https://doi.org/10.1145/3644116.3644294]
- Zhang H, Wang X, Meng Z, Jia Y, Xu D. Qibo: A Large Language Model for Traditional Chinese Medicine. arXiv preprint arXiv:240316056, . 2024.
- Kang B, Kim J, Yun T-R, Kim C-E. Prompt-RAG: Pioneering Vector Embedding-Free Retrieval-Augmented Generation in Niche Domains, Exemplified by Korean Medicine. arXiv preprint arXiv:240111246, . 2024.
-
Yang G, Shi J, Wang Z, Liu X, Wang G. TCM-GPT: Efficient Pre-training of Large Language Models for Domain Adaptation in Traditional Chinese Medicine. arXiv preprint arXiv:231101786, . 2023.
[https://doi.org/10.1016/j.cmpbup.2024.100158]
-
Tan Y, Zhang Z, Li M, Pan F, Duan H, Huang Z, et al. MedChatZH: A tuning LLM for traditional Chinese medicine consultations. Computers in Biology and Medicine. 2024;172:108290.
[https://doi.org/10.1016/j.compbiomed.2024.108290]
- Yang T, Wang X-Y, Zhu Y, Hu K-F, Zhu X-F. Research Ideas and Methods of Intelligent Diagnosis and Treatment of Traditional Chinese Medicine Driven by Large Language Model. Journal of Nanjing University of Traditional Chinese Medicine. 2023;39(10):967-71.
-
Pu H, Mi J, Lu S, He J, editors. RoKEPG: RoBERTa and Knowledge Enhancement for Prescription Generation of Traditional Chinese Medicine. 2023 IEEE International Conference on Bioinformatics and Biomedicine (BIBM); 2023: IEEE.
[https://doi.org/10.1109/BIBM58861.2023.10385636]
- Wang X, Yang T, Hu K. Research on personalized prescription recommendation of traditional Chinese medicine based on large language pre-training model. Chinese Archives of Traditional Chinese Medicine. 1-14.
-
Li Y, Peng X, Li J, Zuo X, Peng S, Pei D, et al. Relation Extraction Using Large Language Models: A Case Study on Acupuncture Point Locations. arXiv preprint arXiv:240405415, . 2024.
[https://doi.org/10.1093/jamia/ocae233]
-
Zhou Z, Yang T, Hu K, editors. Traditional Chinese Medicine Epidemic Prevention and Treatment Question-Answering Model Based on LLMs. 2023 IEEE International Conference on Bioinformatics and Biomedicine (BIBM); 2023 5-8 Dec. 2023.
[https://doi.org/10.1109/BIBM58861.2023.10385748]
-
Liu C, Sun K, Zhou Q, Duan Y, Shu J, Kan H, et al. CPMI-ChatGLM: parameter-efficient fine-tuning ChatGLM with Chinese patent medicine instructions. Scientific Reports. 2024;14(1):6403.
[https://doi.org/10.1038/s41598-024-56874-w]
-
Wang Z, Li K, Ren Q, Yao K, Zhu Y, editors. Traditional Chinese Medicine Formula Classification Using Large Language Models. 2023 IEEE International Conference on Bioinformatics and Biomedicine (BIBM); 2023 5-8 Dec. 2023.
[https://doi.org/10.1109/BIBM58861.2023.10385776]
- Zhang J, Yang S, Liu J, Huang Q. AIGC Empowering the Revitalization of Traditional Chinese Medicine Ancient Books: A Study on the Construction of the Huang-Di Large Language Model. Library Tribune. 1-13.
- Tonmoy S, Zaman S, Jain V, Rani A, Rawte V, Chadha A, et al. A comprehensive survey of hallucination mitigation techniques in large language models. arXiv preprint arXiv:240101313, . 2024.
-
Zhang D, Yu Y, Li C, Dong J, Su D, Chu C, et al. Mm-llms: Recent advances in multimodal large language models. arXiv preprint arXiv:240113601, . 2024.
[https://doi.org/10.18653/v1/2024.findings-acl.738]
-
Gou J, Yu B, Maybank SJ, Tao D. Knowledge distillation: A survey. International Journal of Computer Vision. 2021;129:1789-819.
[https://doi.org/10.1007/s11263-021-01453-z]
-
Elbadawi M, Li H, Basit AW, Gaisford S. The role of artificial intelligence in generating original scientific research. International Journal of Pharmaceutics. 2024;652:123741.
[https://doi.org/10.1016/j.ijpharm.2023.123741]