
인공지능시대의 경혈 주치 연구를 위한 제언
Ⓒ The Society of Pathology in Korean Medicine, The Physiological Society of Korean Medicine
Abstract
Artificial intelligence technology sheds light on new ways of innovating acupuncture research. As acupoint selection is specific to target diseases, each acupoint is generally believed to have a specific indication. However, the specificity of acupoint selection may be not always same with the specificity of acupoint indication. In this review, we propose that the specificity of acupoint indication can be inferred from clinical data using reverse inference. Using forward inference, the prescribed acupoints for each disease can be quantified for the specificity of acupoint selection. Using reverse inference, targeted diseases for each acupoint can be quantified for the specificity of acupoint indication. It is noteworthy that the selection of an acupoint for a particular disease does not imply the acupoint has specific indications for that disease. Electronic medical record includes various symptoms and chosen acupoint combinations. Data mining approach can be useful to reveal the complex relationships between diseases and acupoints from clinical data. Combining the clinical information and the bodily sensation map, the spatial patterns of acupoint indication can be further estimated. Interoperable medical data should be collected for medical knowledge discovery and clinical decision support system. In the era of artificial intelligence, machine learning can reveal the associations between diseases and prescribed acupoints from large scale clinical data warehouse.
Keywords:
Acupoint indication, Artificial intelligence, Big data, Data mining, Machine learning서 론
침술은 한의학에서 가장 대표적인 치료도구로서, 침을 이용하여 경혈이라 불리는 인체의 특정 부위를 자극하여 효과를 나타내는 치료법이다1). 경락시스템은 경혈 주치를 시스템적으로 설명하는 한의학 이론으로, 질병과 침 자극 부위인 경혈과의 연계성을 설명하는 모델이다2). 침구의학은 환자의 몸에서 나타나는 질병 및 증상의 패턴을 관찰하여, 가장 효과적인 자극 위치를 선정하고 적절한 자극 방법을 수행하는 것을 주된 목적으로 한다. 임상연구를 통해 다양한 질환에 대한 침 치료의 유효성을 밝히려는 시도들이 진행되고 있다3). 한의과대학에서는 주로 경혈학과 침구학 과목에서 경혈 주치와 경혈 선혈의 방식과 관련된 침술 원리를 학습한다. 침구학에서는 특정한 질환 혹은 증상 패턴에 기반하여 최적 경혈을 선혈하는 원리를 학습한다4). 경혈학에서는 사용되는 경혈의 정확한 위치와 함께 경혈이 주로 치료할 수 있는 증상, 즉 ‘경혈 주치’에 대하여 학습한다5). 한의학 고전문헌에서도 (1) 경혈 선혈 측면, 즉, 특정 질환에 어떤 경혈을 주로 사용한다는 기술과 (2) 경혈 주치 측면, 즉 특정 경혈은 어떤 질환을 주로 치료한다는 기술이 함께 나타난다. 임상에서는 환자의 대상 질환에 대해 특정 경혈을 사용한 사례를 기반으로 대상 질환과 사용된 경혈의 관계에 대한 정보를 학습한다. 관찰된 임상경험들을 바탕으로 특정 질환에 대해 어떤 경혈을 사용하는 것이 효과적인지 결과에 기반하여, 특정 질환치료에 가장 유용한 경혈을 기록하고 이러한 정보가 전해져서 경혈 선혈 근거를 이루게 된다6). 이러한 특정 질환과 경혈과의 관계를 기반으로, 경혈이 치료할 수 있는 질환 특징들이 경혈 주치특성으로 교육된다7).
인공지능(artificial intelligence: AI) 기술이 의학분야에 도입되면서 전통의학분야에서도 이를 적용하고 새로운 지식 발굴에 활용하고 있다8,9). 데이터마이닝(data mining)방법을 통해 질환별로 경혈 선혈 원리를 규명하고자하는 시도들이 진행되고 있다10). 초기 연구들에서는 고전문헌에 기술된 선혈 패턴을 분석한 시도가 진행되어, 예를 들어 前秦시대부터 淸代에 이르는 침구문헌에서 설사의 침 치료를 위해 천추, 신궐, 관원, 대장수가 주로 선혈된다는 사실을 밝혔다11). 이후 연구들에서는 미국국립의학정보원(Pubmed) 및 중국학술정보원(Chinese National Knowledge Infrastructure: CNKI) 등 다양한 데이터베이스를 통해, 긴장형 두통의 침 치료를 위해 선혈되는 패턴을 분석하여, 풍지, 백회, 태양, 합곡, 태충이 주로 선혈된다고 제시하였다12). 또한, 요통의 침 치료를 위해 선혈되는 패턴을 분석하여, 신수, 대장수, 기해수, 위중, 곤륜, 환도가 주로 선혈됨을 확인하였다13,14). 또한, 실사용데이터(real world data: RWD)를 이용하여, 전자의무기록 분석을 통해 뇌성마비의 침 치료 패턴을 분석하여, 어떤 경혈 조합이 주로 사용되는지 제시하였다15). 기계학습(machine learning: ML)을 적용하여 침구의학에서 경혈 선혈 방식을 이해하려는 시도들이 증가하고 있다16). 최근, 인공신경망(artificial neural network: ANN)을 적용하여, 환자의 증상과 사용된 경혈 관계를 학습하여, 새롭게 주어진 환자의 증상을 치료하기 위해 어떤 경혈이 선혈되는지 성공적으로 예측하였다17). 이러한 방식으로 특정 질환에 어떤 경혈이 주로 선혈되는지 확인하고, 이를 통해 경혈 선혈의 특이성을 갖는다는 것을 알 수 있다.
베이지안 이론(Bayesian theorem)에 따르면, 관찰된 데이터(observed data)에 대한 확률적 모델(statistical model)에서 사후확률(posterior probability)을 추론한다18). 침 치료에서 임상에서 관찰한 현상 중에서, 환자의 대상 질환과 한의사의 침치료에 적용한 경혈과의 비선형적 관계를 학습하게 된다. 특정 질환에 대해 어떤 경혈이 특이적으로 선혈되는지 살펴보고, 순행성 추론(forward inference)을 통해 특정 질환에 대한 경혈 선혈의 특이성(specificity for acupoint selection)을 판단할 수 있다19). 반대로, 특정 경혈에 대해 어떤 질환이 특이적으로 관련되는지를 살펴보고, 역행성 추론(reverse inference)을 통해 특정 경혈에 대한 경혈 주치의 특이성(specificity for acupoint indication)을 알 수 있다20). 일반적으로 순행성 추론과 역행성 추론을 통한 대상 질환과 경혈과의 관계가 크게 달라지지는 않는다. 예를 들어, 역류성식도염 치료를 위해 다른 경혈에 비해 중완이 특이적으로 선혈되고, 중완은 다른 질환에 비해 역류성식도염을 특이적으로 주치한다. 그러나, 순행성 추론의 결과와 역행성 추론의 결과가 항상 일치하는 것은 아니다. 예를 들어, 공황장애 치료를 위해 다른 경혈에 비해 내관이 특이적으로 선혈되지만, 내관은 다른 질환에 비해 공황장애를 특이적으로 주치하는 것은 아니다20). 그러므로, 순행성 추론은 특정 질환에 대해 어떤 경혈이 특이적으로 선혈되는지를 말할 뿐이어서, 이를 통해 경혈 주치의 특이성을 결정하는 것은 논리적인 문제를 발생시키게 된다. 경혈 주치의 특이성은 역행성 추론을 통해 확인될 수 있게 된다20). 따라서, 경혈 선혈의 원리를 통해 판단한 특정 질환과 경혈의 관계가 경혈 주치와 동일하지 않을 수 있다는 점을 인식할 필요가 있다.
고전문헌 및 임상데이터를 기반으로 질환과 경혈의 관계를 이해하기 위한 접근이 진행되어 왔다. 본 연구에서는 순행성 추론을 통한 침구학에서 경혈 선혈의 특이성 측면과 역행성 추론을 통한 경혈학에서 경혈 주치의 특이성 측면을 구분하여 이해할 필요성을 제안한다. 아울러 문헌 정보와 임상 정보를 기반으로 데이터마이닝 방식을 이용하여 현재까지 진행된 경혈 선혈의 특이성과 경혈 주치의 특이성을 규명하고자 한 연구를 소개하고자 한다. 아울러, 향후 한의학 정보의학 분야에서, 전자의무기록 시스템과 인공지능을 기반으로 경혈 주치특성에 대한 연구 방안을 제안하고자 한다.
본 론
1. 경혈 선혈의 특이성과 경혈 주치의 특이성
『鍼灸大成』의 卷9 「治症總要」에서 각 질환에서 어떤 경혈을 선혈하는지에 대한 기술(경혈선혈의 특이성)과 卷7에 포함된 「十二經脈穴主治」에서 기록된 각 경혈이 어떤 증상을 치료하는 지에 대한 기술(경혈주치의 특이성)을 확인할 수 있다. [예시 1]에서 심흉동통을 치료를 위해 내관이 선혈되고, 내관이 치료할 수 있는 주치증상으로 심통이 포함되어 있다. 특정 질환에 어떤 경혈이 특이적으로 선혈되는지 특성을 바탕으로 경혈의 주치특성이 추론될 수 있다. 대부분의 경우, 경혈 선혈의 특이성과 경혈 주치의 특이성이 일치한다고 볼 수 있다. 반면, [예시 2]에서 대변비결을 치료를 위해 조해가 선혈되지만, 조해가 치료할 수 있는 주치증상으로 대변비결이 포함되어 있지 않다. 고전문헌에서 경혈 선혈과 경혈 주치에 대해 치밀하게 기록하지 못한 측면이 있을 수 있지만, 이렇듯 경혈 선혈의 특이성과 경혈 주치의 특이성이 항상 일치하지는 않을 수 있다는 점을 시사한다.
데이터마이닝을 통한 특정 질환에 어떤 경혈이 특이적으로 선혈되는지 살펴본 연구를 통해, 경혈 선혈의 특이성을 확인할 수 있다. 데이터마이닝 방법을 적용하여, 고전문헌 및 임상연구 데이터를 분석하여 만성안정형협심증(chronic stable angina pectoris), 역류성식도염(gastroesophageal reflux disease), 월경통(dysmenorrhea), 설사(diarrhea) 질환에 어떤 경혈이 주로 선혈되는지 살펴보았다(Fig. 1). 만성안정형협심증 침 치료에 내관, 태연, 족삼리가 주로 사용되고, 역류성식도염 침 치료를 위해 중완, 족삼리, 내관이 주로 사용되었다21,22). 월경통 침 치료를 위해 삼음교, 기해, 관원이 주로 사용되고, 설사 침 치료를 위해 천추, 신궐, 관원, 대장수가 주로 사용되었다11,23). 이러한 순행성 추론 방식을 통해, 경혈 선혈의 특이성, 즉, 특정 질환에 어떤 경혈이 특이적으로 선혈되는지 알 수 있다.
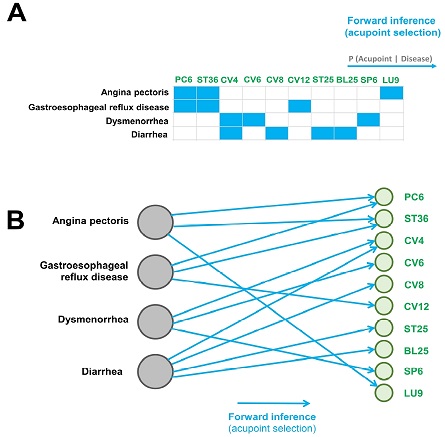
Specificity of acupoint selection by forward inference. A: Forward inference for acupoint selection. The most frequently used acupoints to treat chronic stable angina pectoris were PC6, LU9, and ST36 acupoints. The most frequently used acupoints to manage gastroesophageal reflux disease were CV12, ST36, and PC6. The most frequently used acupoints to treat dysmenorrhea were SP6, CV6, and CV4 acupoints. The most frequently used acupoints to manage diarrhea were ST25, CV8, CV4, and BL25. B: Non-linear complex relationships between the diseases and the acupoints. The diseases include chronic stable angina pectoris, gastroesophageal reflux disease, dysmenorrhea, and diarrhea (grey circle). The acupoints include PC6, ST36, CV4, CV6, CV8, CV12, ST25, BL25, SP6, and LU9 (green circle). The line represents one-to-multiple relationships from diseases to acupoints.
순행성 추론(forward inference)을 통해 특정 질환에 대해 어떤 경혈이 특이적으로 선혈되는지 살펴보고, 특정 질환에 대한 경혈 선혈의 특이성(specificity for acupoint selection)을 확인할 수 있다. 반대로, 역행성 추론(reverse inference)을 통해 특정 경혈에 대해 어떤 질환이 특이적으로 관련되는지 살펴보고, 특정 경혈에 대한 경혈 주치의 특이성(specificity for acupoint indication)을 확인할 수 있다.
순행성 추론에 따른 경혈 선혈의 특이성과 역행성 추론에 따른 경혈 주치의 특이성은 동일하지 않을 수 있다. 이에 대한 이해를 돕기 위해, 사전연구에서 발표한 30개의 질환에 선혈된 경혈을 예시로 설명하고자 한다24). 예를 들어, 고혈압 질병과 족삼리, 태충, 해계, 행간, 부류, 풍지, 곡지 경혈과의 관계를 살펴보면, (1) 고혈압 치료를 위해 족삼리와 태충은 특이적으로 선혈되어, 순행성 추론을 통해 고혈압 치료에 있어 족삼리와 태충은 선혈의 특이성을 지닌다(경혈 선혈의 특이성). (2) 고혈압 치료를 위해 해계, 행간, 부류가 특이적으로 선혈되지는 않지만, 역행성 추론을 통해 해계, 행간, 부류는 고혈압 치료 경혈 주치의 특이성을 지닌다(경혈 주치의 특이성). (3) 고혈압 치료를 위해 풍지와 곡지는 특이적으로 선혈되어, 순행성 추론을 통해 고혈압 치료에서 풍지와 곡지는 선혈의 특이성을 지닌다. 또한. 역행성 추론을 통해 풍지와 곡지는 고혈압 치료의 경혈 주치의 특이성도 지닌다(경혈 선혈의 특이성과 경혈 주치의 특이성)(Fig. 2).
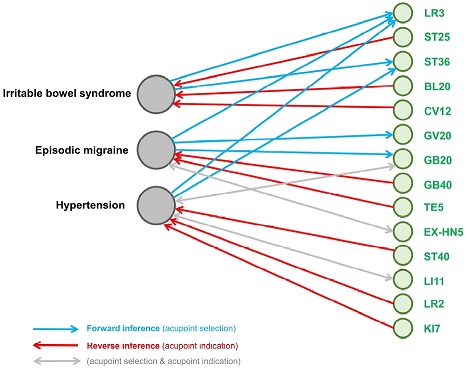
Forward inference and reverse inference in acupuncture medicine. Example of forward inference for specificity of acupoint selection and reverse inference for specificity of acupoint indication. The ST36 and LR3 acupoints can be selected specifically for hypertension, but ST36 and LR3 have no specific indication for the treatment of hypertension (blue line: forward inference). The ST40, LR2, and KI7 acupoint are not selected specifically for hypertension, but ST40, LR2, and KI7 have a specific indication for hypertension (red line: reverse inference). The GB20 and LI11 acupoint can be selected specifically for hypertension, and they have a specific indication for the treatment of hypertension (grey line: forward and reverse inference). The diseases include irritable bowel syndrome, episodic migraine and hypertension (grey circle). The acupoints include LR3, ST25, ST36, BL20, CV12, GV20, GB20, GB40, TE5, EX-HN5, ST40, LI11, LR2, and KI7 (green circle).
특정 질환에 어떤 경혈이 특이적으로 선혈되는지 추론하는 방식을 통해 경혈 선혈의 특이성을 확인할 수 있다. 그러나, 특정 경혈이 어떤 질환을 특이적으로 치료하는 경혈 주치의 특이성은 역행성 추론 과정이 필요하고, 이러한 과정에서 경혈 선혈의 특이성과 경혈 주치의 특이성이 일치하지 않는 경우가 발생할 수 있다. 경혈 주치의 특이성을 확인하기 위해서는 역행성 추론 과정을 통해 주어진 경혈에 대해 어떤 질환이 특이적으로 관여하는지에 대한 분석이 필요하다.
2. 고전문헌에서 경혈 주치특성 분석
데이터마이닝을 통해 한의학 대표적 의서, 허준의 『東醫寶鑑』과 허임의 『鍼灸經驗方』을 대상으로 질병의 부위와 경혈과의 관계 분석하여, 특정 경혈이 어떤 질병을 치료하는 특성을 지니는 지를 확인하였다. 즉, 역행성 추론을 통해 경혈 주치특성을 추출하였다.
『東醫寶鑑』 「外形篇」에서 [頭], [面], [眼], [耳] 등 25개 문 중에서 신체부위 질병에 대한 특성이 명확한 14개 질병 부위로 취합하고, 이를 치료하기 위해 사용한 경혈들의 소속 경맥을 통합하여 14개 경맥 정보로 취합하였다25). 데이터마이닝 분야에서 주로 사용하는 TF-IDF(Term Frequency-Inverse Document Frequency) 정규화 방식을 적용하여, 특정 단어가 특정 문서와 얼마나 연관되는지 정규화하여 나타낸다. 각 신체부위 질병에 어떤 경맥의 경혈이 선혈되는 지를 관찰하는 경우, 고전 문헌 데이터에서 순행성추론의 과정을 통해 경혈 선혈 확률을 파악할 수 있게 된다. 반대로, 어떤 경맥의 경혈들이 주로 치료할 수 있는 신체부위 질병인지 관찰하는 경우, 고전 문헌 데이터에서 역행성 추론 과정을 통해 경혈(혹은 경맥)의 주치특성을 파악할 수 있게 된다.
해당 연구에서 수삼음경 경맥의 경혈은 乳胸, 咽喉, 手 부위 주치특성이 높고, 수삼양경 경맥의 경혈은 手, 耳, 鼻 부위 주치특성이 높았다. 족삼음경 경맥의 경혈은 乳胸, 脇, 足 부위 주치특성이 높고, 족삼양경 경맥의 경혈은 足, 眼, 頭, 腰背, 乳胸, 脇 부위 주치특성이 높았다. 이상에서 수족 삼음삼양경의 경혈들과 신체 주치부위와 연관성을 신체도에 매핑하여 가시화하였고, 경맥유주 특성과 경맥내에 존재하는 경혈의 주치특성이 일치하는 현상을 발견할 수 있었다.
『鍼灸經驗方』의 43개 문 중에서, [頭面], [耳], [目], [鼻] 등 질병 부위에 대해 기록한 13개 문을 분석하여 20개 질병 부위를 취합하였다26). 그리고, 해당 질환 부위를 치료하기 위해 사용된 110개의 경혈 중에서 원혈을 추출하였다. 마찬가지로 TF-IDF 정규화 방식을 적용하여, 특정 경혈(원혈)이 특정 질병과 얼마나 연관되는지를 정규화하였다.
해당 연구에서 수삼음경의 원혈인 태연, 신문, 대릉의 경우, 心胸과 上肢의 주치특성이 높았고, 수삼양경의 원혈인 합곡과 완골의 경우, 頭, 面, 耳, 目, 口, 齒, 鼻, 上肢의 주치특성이 높았다. 족삼음경의 원혈인 태백, 태계, 태충의 경우, 胸, 腹, 下肢의 주치특성이 높았다. 원혈의 주치증상 신체부위는 해당 원혈이 속하는 경맥의 유주와 매우 높은 연관을 지님을 알 수 있다. 원혈의 주치특성의 신체부위의 패턴을 살펴보면, 경락 유주노선은 경혈 주치특성을 설명하는 임상적 의의가 있다고 볼 수 있다(Fig. 3).
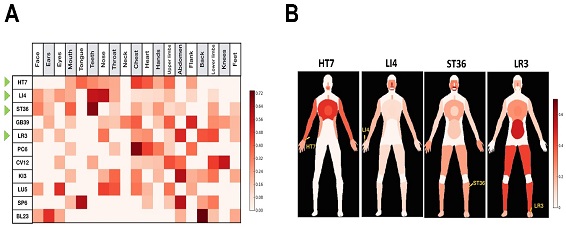
Spatial patterns of the indications of acupoints using data mining. A: Data mining methods were applied to analyze the characteristics of acupoint indication in the classic Korean medicine literature. The disease sites are presented on the x-axis of the array and the representative acupoints are presented on the y-axis of the array. B: The spatial patterns of the acupoint indications were visualized on a human body template (HT7, LI4, ST36, and LR3).
3. 임상연구 데이터베이스에서 경혈 주치특성 분석
증례보고 혹은 임상연구에서 특정 질환을 치료하기 위하여 사용된 경혈의 빈도에 기반하여, 특정 질환과 경혈과의 연관성을 분석하여 경혈 선혈의 특이성과 경혈 주치의 특이성을 추출하였다. 즉 순행성 추론을 통해 특정 질환에 어떤 경혈이 선혈되는지 확인하고, 역행성 추론을 통해 특정 경혈이 어떤 질환을 치료하는지 확인하였다.
증례보고 10개 주요 질환을 대상으로, 80명 한의사들에게 모의 침구치료에 사용된 경혈 정보를 추출하였다20). 먼저 순행성 추론을 통해 특정 질환에 어떤 경혈이 특이적으로 선혈되는지 확인하였다. 양성돌발두위현훈(benign paroxysmal positional vertigo, BPPV) 치료에는 족삼리, 합곡, 중완이 주로 사용되고, 추간판탈출증(lumbar herniated intervertebral disc) 치료에는 신수, 환도, 요양관이 주로 사용되었다. 특정 질환에 어떤 경혈이 특이적으로 선택되는지 분석하여, 경혈 선혈의 특이성을 제시하는 결과로 볼 수 있다. 반면에, 역행성 추론을 통해 특정 경혈이 어떤 질환의 주치특성을 지니는지 확인하였다. 음곡은 섬유근통을 치료하는 경혈 주치의 특이성을 보이고, 신수는 추간판탈출증을 치료하는 경혈 주치의 특이성을 지닌다.
데이터마이닝을 통해 질병과 경혈의 관계를 분석하기 위해, 코크레인 데이터베이스(Cochrane Database of Systematic Reviews: CDSRs)에서 30개 질환을 대상으로 침구 치료에 사용된 경혈 정보를 추출하였다24). 먼저 순행성 추론을 통해 특정 질환에 어떤 경혈이 특이적으로 선혈되는지 확인하였다. 우울증 침 치료에 백회, 인당이 주로 사용되고, 생리통 침 치료에는 삼음교, 관원, 지기가 주로 사용되고, 불면증 침 치료에 신문, 백회가 주로 사용된다(Fig. 4A). 이는 특정 질환에 어떤 경혈이 특이적으로 관련되는지 분석하여, 경혈 선혈의 특이성을 제시하는 결과로 볼 수 있다. 반면에 역행성 추론을 통해 특정 경혈이 어떤 질환의 주치특성을 지니는지 확인하였다. 삼음교는 우울증과 생리통 치료의 경혈 주치의 특이성을 보이고, 합곡은 우울증, 유도분만, 수정능력저하증 치료의 경혈 주치의 특이성을 보인다(Fig. 4B).
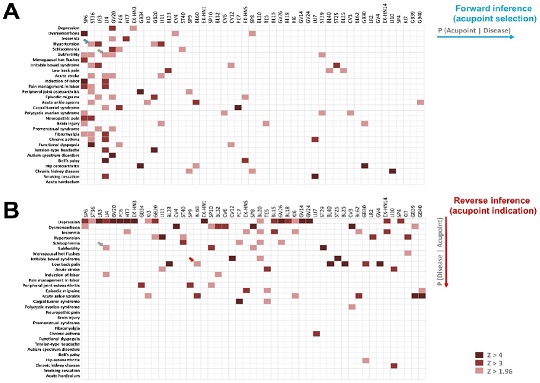
Acupoint selection using forward inference and acupoint indication using reverse inference. A: Forward inference of the specificity of acupoint selection. The Z scores illustrate how frequently each acupoint was used for each disease compared to the overall mean prescription frequency for that disease. B: Reverse inference of the specificity of acupoint indications. The Z scores illustrate how frequently each disease was associated with each acupoint compared to the overall mean disease for that acupoint. Probability values were Z-transformed for the assessment of statistical significance (Z>1.96). Pattern 1: The LI4 acupoint can be selected specifically for subfertility, and it has a specific indication for the treatment of subfertility (grey arrow). Pattern 2: The ST36 acupoint can be selected specifically for hypertension, but ST36 has no specific indication for the treatment of hypertension (blue arrow). Pattern 3: The BL60 acupoint is not selected specifically for low back pain, but BL60 has a specific indication for low back pain (red arrow).
4. 역행성 추론을 통해 경혈 주치특성 탐색과정에서 고려사항
순행성 추론과 역행성 추론을 통해 특정 질환과 경혈과의 관계를 살펴볼 때, 다음 세 가지 패턴이 나타날 수 있다24). 첫째, 순행성 추론과 역행성 추론이 일치하는 경우이다. 예를 들어, 수정능력저하증을 치료하기 위해 합곡은 경혈 선혈의 특이성을 보이고, 또한 합곡은 수정능력저하증 치료의 경혈 주치의 특이성을 보이는 경우이다. 둘째, 순행성 추론은 성립하지만, 역행성 추론이 성립하지 않는 경우이다. 예를 들어, 고혈압을 치료하기 위해 족삼리는 경혈 선혈의 특이성을 보이지만, 반대로 족삼리는 고혈압 치료의 경혈 주치의 특이성을 보이지는 않는 경우이다. 셋째, 순행성 추론은 성립하지 않지만, 역행성 추론은 성립하는 경우이다. 예를 들어, 요통 치료에 곤륜은 경혈 선혈의 특이성을 보이지는 않지만, 반대로 곤륜은 요통 치료의 경혈 주치의 특이성을 보이는 경우이다(Fig. 4).
경혈 선혈의 특이성과 경혈 주치의 특이성이 항상 일치하는 것은 아니다라는 사실을 주지할 필요가 있다. 순행성 추론을 통해 특정 질환에 어떤 경혈이 특이적으로 선혈되는지 패턴을 확인할 수 있지만, 이러한 방식을 통해 경혈 주치의 특이성이 일치할 것으로 결론짓는 것은 논리적 오류를 발생시킬 수 있다. 경혈 주치의 특이성 확인은 특정 경혈에 대해 어떤 질환이 특이적인 관계를 가지는지 확인하는 역행성 추론을 통해서 가능하다. 경혈 주치의 특이성은 경혈 선혈의 경험 등을 통해 질병과 경혈의 선혈 관계를 바탕으로 추론될 수 있는 것이지, 경혈 주치특성을 기반으로 경혈 선혈이론이 만들어 진 것으로 이해해서는 안 된다.
경혈 선혈의 특이성을 이야기할 때, 합곡과 족삼리와 같이 여러 질환에 다빈도로 사용되는 경혈의 경우, 어떤 질환에 특이적으로 이러한 경혈이 사용된다고 이야기하기 어렵다19). 즉, 합곡, 족삼리와 같이 다양한 질환에 범용적으로 사용되는 경우, 특정 질환 치료에 특이성을 지닌다고 보기 어렵다. 다빈도 경혈의 경우는 경혈 선혈의 공통성이 높다고 말할 수는 있지만, 경혈 선혈의 특이성이 높다고 말하기 어려운 측면이 있다. 마찬가지로 경혈 주치의 특이성을 이야기할 때, 특정 데이터베이스에 많이 포함된 질환 혹은 특정 의료기관에 많이 포함된 질환의 경우, 어떤 경혈이 이러한 질환을 특이적으로 주치하는 특성을 지닌다고 보기 어렵다. 다빈도 질환의 경우 질환의 높은 발생 빈도로 인해 여러 경혈에서 주치증상으로 나타날 수 있으므로, 경혈 주치의 특이성이 높다고 할 수 없다.
코크레인 데이터베이스 30개 질환 대상 경혈 주치특성 분석 연구에서 우울증과 생리통의 연구의 빈도가 높은 것으로 인해, 역행성 추론을 할 경우 주치특성이 높게 나오는 경향이 생긴다. 역행성 추론을 통해 경혈 주치의 특이성을 추론할 경우, 해당 질병의 빈도에 영향을 받기 때문에 질병의 빈도에 대한 값을 보정할 필요가 있다. 베이지안 계수(Bayesian factor)를 고려하여 역행성 추론을 통해 경혈 주치의 특이성을 추론하면, 이러한 다빈도 질환으로 인한 부분은 보정 될 수 있다. 베이지안 계수를 통해 확인한 경혈 주치를 분석한 경우, 신수-요통, 대장수-요통, 곤륜-족과관절염 등의 근위 부위 주치특성과 곤륜-요통, 신문-불면증, 수삼리-만성신질환 등의 원위 부위 주치특성을 확인할 수 있다24).
5. 경혈 주치특성 분석 연구를 위한 제언
한의학 분야에서 임상 데이터웨어하우스(clinical data warehouse)를 통해, 구조화된 전자의무기록데이터(structured medical record data)를 적용하여 새로운 의학지식 발견과 임상 의사결정지원체계(clinical decision support system: CDSS)를 구축하고 있다27). 구축된 데이터 베이스에서 환자의 질환 정보 및 경혈 정보 데이터의 추출-변환-적재(extraction-transformation-loading: ETL) 과정을 거쳐, 유용한 임상 정보를 통합하고 분석이 가능하다28).
실사용데이터에 기반하여 경혈 선혈의 특이성과 경혈 주치의 특이성을 추출할 수 있다. 이를 위해서는 전자의무기록에 포함되는 환자의 주소증, 상병명, 변증정보 등 질환 정보와 사용된 경혈 정보가 공유되는 시스템이 필요하다29). 한의원 및 한방병원에서 침구 치료과정에서 수반되는 한의학적 진단 과정을 포괄하고 통합할 수 있는 진료기록 시스템을 구축하고, 수집된 데이터의 정제, 가공, 시각화할 수 있는 분석시스템 개발이 함께 진행되어야 한다17). 이러한 통합된 의료정보를 기반으로, 순행성 추론을 통해 특정 질환에 어떤 경혈이 선혈되는지 즉, ‘경혈 선혈의 특이성’을 분석할 수 있고, 아울러 역행성 추론을 통해 특정 경혈이 어떤 질환에 특이적으로 선혈되는지 즉, ‘경혈 주치의 특이성’을 분석할 수 있다.
전자의무기록을 작성하는 경우, 환자 병소(病所)에 대한 정보를 입력 받고, 이를 치료하기 위해 사용된 경혈과 환자의 증상 부위와 연관성을 분석하는 것이 필요하다. 특정 질환과 경혈과의 관계를 분석하여, 경혈 주치특성을 추론할 수 있는데, 이러한 주치특성은 신체공간상의 특정한 패턴을 지닐 수 있다. 침구 치료 원리를 제안하는 경락시스템은 질병의 부위와 경혈 선혈의 원리를 가이드 해준다. 최근연구에서, 75명 통증 환자의 통증 부위 정보를 신체감각지도(bodily sensation map) 시스템을 통해 획득하고, 이를 치료하기 위해 선혈된 경혈 정보와 결합하여, 특정 경혈의 주치 증상 부위와의 연관성을 분석하였다30). 전자진료기록 시스템과 함께 신체감각지도를 활용하여, 질병 발생 부위 정보를 통합적으로 수집, 정제, 분석하면, 경락시스템의 유용성을 데이터에 기반하여 확인할 수 있을 것이다(Fig. 5).
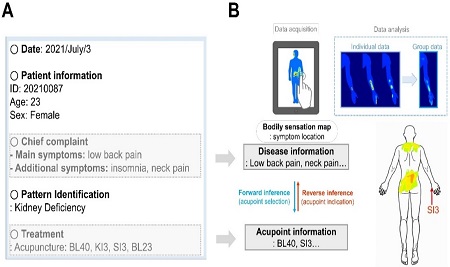
Acupoint indication using artificial intelligence. Electronic medical record includes symptoms, pattern identification, and chosen acupoints combination. Interoperable medical data should be collected for medical knowledge discovery and clinical decision support system. Data mining and machine learning methods can be applied to reveal the associations between diseases and prescribed acupoints. Combining the clinical information and the bodily sensation map, the spatial patterns of acupoint indication can be further estimated.
기존 문헌연구 혹은 임상데이터의 경우, 환자의 질병과 경혈 선혈 정보만을 포함하고 있다. 단순히 진료기록을 통합하는 것이 아니라, 환자의 치료 경과 및 치료효과 정보를 반영하여, 유효한 침구 치료의 방식과 그렇지 않은 침구 치료의 방식을 구분할 필요가 있다. 즉, 침구 치료 효과가 반영된 경혈 주치의 특이성을 추출하기 위해서는 환자 질병 호전도를 정량적으로 포괄하는 데이터가 반영되어야 한다. 단순히 경혈 선혈 사용빈도를 대상으로 경혈 주치의 특이성을 이야기하는 것 아니라, 질병과 경혈의 관계를 학습하는 과정에서 환자의 질병 호전도가 일정 수준 이상 정보만을 포함하여 분석하는 방식을 적용할 수 있다. 아울러, 실사용데이터를 활용하는 경우, 동반질환에 대한 고려가 필요하고, 다양한 치료가 동시에 수행되는 것을 고려하여 분석하는 것이 필요하다.
결 론
이상에서 고전 문헌과 현대 임상연구 데이터에 포함된 질병과 경혈의 관계를 기반으로, 순행성 추론을 통한 경혈 선혈의 특이성과 역행성 추론을 통한 경혈 주치의 특이성을 확인하였다. 임상현장에서 침구 치료가 수행되는 과정에서 나타나는 질병과 경혈 선혈의 방식을 기반으로 경혈 주치특성을 확인하기 위해, 전자의무기록 시스템을 통한 데이터의 정제, 가공, 시각화할 수 있는 분석시스템 개발이 필요하다. 환자의 치료 효과가 반영되고, 질병 발생 부위 정보를 통합적으로 분석할 수 있는 시스템을 통해, 경혈의 주치특성을 추출할 수 있다. 다양한 형태의 의료정보가 통합되는 빅데이터 시대에 경혈 선혈방식, 그리고 경혈 주치특성을 연구하는 방식도 함께 발전되어 나가야 할 것을 제안한다.
Acknowledgments
This research was supported by Korea Institute of Oriental Medicine (KSN1812181) and Basic Science Research Program through the National Research Foundation of Korea (NRF) funded by the Ministry of Science, ICT & Future Planning (No. 2021R1F1A1046705).
References
-
Chae Y, Chang DS, Lee SH, Jung WM, Lee IS, Jackson S, et al. Inserting needles into the body: a meta-analysis of brain activity associated with acupuncture needle stimulation. J Pain. 2013;14(3):215-22.
[https://doi.org/10.1016/j.jpain.2012.11.011]
-
Chae Y. Past, present, and the future of understanding the entity of the meridian system. J Physiol & Pathol Korean Med. 2016;30(6):402-11.
[https://doi.org/10.15188/kjopp.2016.12.30.6.402]
-
Jeon SH, Lee IS, Lee H, Chae Y. A bibliometric analysis of acupuncture research trends in clinical trials. Kor J Acu. 2019;36(4):281-91.
[https://doi.org/10.14406/acu.2019.030]
-
Kye K, Kim B. A study on methods of acupuncture points combination and hierarchy concept of acupuncture prescription in Korean medicine. J Korean Med. 2020;41(3):9-21.
[https://doi.org/10.13048/jkm.20022]
-
Kim M, Han CH. A survey on the educational status of basic Korean medicine and basic medical science in colleges of Korean medicine in 2020. J Korean Med. 2020;41(3):98-124.
[https://doi.org/10.13048/jkm.20028]
-
Park IS, Jung WM, Lee YS, Hahm DH, Park HJ, Chae Y. Characterization of Five-Shu acupoint pattern in Saam acupuncture using text mining. Kor J Acu. 2015;32(2):66-74.
[https://doi.org/10.14406/acu.2015.011]
-
Choi DH, Lee SY, Lee IS, Ryu Y, Chae Y. Characteristics of Source acupoints: data mining of clinical trials database. Kor J Acu. 2021;38(2):100-9.
[https://doi.org/10.14406/acu.2021.007]
-
Chu X, Sun B, Huang Q, Peng S, Zhou Y, Zhang Y. Quantitative knowledge presentation models of traditional Chinese medicine (TCM): A review. Artif Intell Med. 2020;103:101810.
[https://doi.org/10.1016/j.artmed.2020.101810]
-
Wang Y, Shi X, Li L, Efferth T, Shang D. The Impact of Artificial Intelligence on Traditional Chinese Medicine. Am J Chin Med. 2021;49(6):1297-314.
[https://doi.org/10.1142/S0192415X21500622]
-
Hwang YC, Lee IS, Ryu Y, Lee MS, Chae Y. Exploring traditional acupuncture point selection patterns for pain control: data mining of randomised controlled clinical trials. Acupunct Med. 2020:964528420926173.
[https://doi.org/10.1177/0964528420926173]
- Su ZW, Ren YL, Zhou SY, Qin HZ, Chen DS, Liu T, et al. Analysis on characteristics of meridians and acupoints of acupuncture and moxibustion for diarrhea in ancient based on data mining. Zhongguo Zhen Jiu. 2013;33(10):905-9.
-
Lu L, Wen Q, Hao X, Zheng Q, Li Y, Li N. Acupoints for Tension-Type Headache: A Literature Study Based on Data Mining Technology. Evid Based Complement Alternat Med. 2021;2021:5567697.
[https://doi.org/10.1155/2021/5567697]
- Lee IS, Jo HJ, Lee SH, Jung WM, Kim SY, Park HJ, et al. Systematic review of selection of acupuncture points for lower back pain. Kor J Acu. 2012;29(4):519-36.
-
Lee IS, Lee SH, Kim SY, Lee H, Park HJ, Chae Y. Visualization of the Meridian System Based on Biomedical Information about Acupuncture Treatment. Evid Based Complement Alternat Med. 2013;2013:872142.
[https://doi.org/10.1155/2013/872142]
- Mou ZJ, He LY, Song HJ, Cheng Q, Liu BY. Rule of point selection in treatment of cerebral palsy in children with acupuncture based on data mining of 1584 electronic medical records. Zhongguo Zhen Jiu. 2021;41(3):355-8.
- Liang J, Han MY, Wang CB, Lu XL, Sun ZR, Yin HN. Research progress in the integration of machine learning and acupunctology. Zhen Ci Yan Jiu. 2021;46(6):460-3.
-
Jung WM, Park IS, Lee YS, Kim CE, Lee H, Hahm DH, et al. Characterization of hidden rules linking symptoms and selection of acupoint using an artificial neural network model. Front Med. 2019;13(1):112-20.
[https://doi.org/10.1007/s11684-017-0582-z]
-
Poldrack RA. Can cognitive processes be inferred from neuroimaging data? Trends Cogn Sci. 2006;10(2):59-63.
[https://doi.org/10.1016/j.tics.2005.12.004]
-
Lee YS, Ryu Y, Yoon DE, Kim CH, Hong G, Hwang YC, et al. Commonality and Specificity of Acupuncture Point Selections. Evid Based Complement Alternat Med. 2020;2020:2948292.
[https://doi.org/10.1155/2020/2948292]
-
Hwang YC, Lee YS, Ryu Y, Lee IS, Chae Y. Statistical inference of acupoint specificity: forward and reverse inference. Integr Med Res. 2020;9(1):17-20.
[https://doi.org/10.1016/j.imr.2020.01.005]
- Sun QH, Li TT, Huang MT, Wang MY, Xiao X, Bai XH. Acupoint selection rules in treating gastroesophageal reflux disease with acupuncture in China based on data mining. Zhongguo Zhen Jiu. 2020;40(12):1374-8.
-
Yu J, Jiang Y, Tu M, Liao B, Fang J. Investigating Prescriptions and Mechanisms of Acupuncture for Chronic Stable Angina Pectoris: An Association Rule Mining and Network Analysis Study. Evid Based Complement Alternat Med. 2020;2020:1931839.
[https://doi.org/10.1155/2020/1931839]
-
Yu S, Yang J, Yang M, Gao Y, Chen J, Ren Y, et al. Application of acupoints and meridians for the treatment of primary dysmenorrhea: a data mining-based literature study. Evid Based Complement Alternat Med. 2015;2015:752194.
[https://doi.org/10.1155/2015/752194]
-
Hwang YC, Lee IS, Ryu Y, Lee YS, Chae Y. Identification of Acupoint Indication from Reverse Inference: Data Mining of Randomized Controlled Clinical Trials. J Clin Med. 2020;9(9).
[https://doi.org/10.3390/jcm9093027]
-
Chae Y, Ryu Y, Jung WM. An analysis of Indications of Meridians in DongUiBoGam using data mining. Kor J Acu. 2019;36(4):292-9.
[https://doi.org/10.14406/acu.2019.034]
-
Jung WM, Lee T, Lee IS, Kim S, Jang H, Kim SY, et al. Spatial Patterns of the Indications of Acupoints Using Data Mining in Classic Medical Text: A Possible Visualization of the Meridian System. Evid Based Complement Alternat Med. 2015;2015:457071.
[https://doi.org/10.1155/2015/457071]
-
Zhou X, Chen S, Liu B, Zhang R, Wang Y, Li P, et al. Development of traditional Chinese medicine clinical data warehouse for medical knowledge discovery and decision support. Artif Intell Med. 2010;48(2-3):139-52.
[https://doi.org/10.1016/j.artmed.2009.07.012]
- Ren YL, Zeng F, Zhao L, Yang J, Liang FR. [Considerations about developing a clinical decision support system for evidence-based diagnosis and treatment of acupuncture-moxibustion]. Zhen Ci Yan Jiu. 2009;34(5):349-52.
- Jung WM, Chae Y, Jang BH. Development of Markup Language for Medical Record Charting: A Charting Language. Stud Health Technol Inform. 2015;216:879.
-
Jung WM, Lee SH, Lee YS, Chae Y. Exploring spatial patterns of acupoint indications from clinical data: A STROBE-compliant article. Medicine (Baltimore). 2017;96(17):e6768.
[https://doi.org/10.1097/MD.0000000000006768]